Understanding and implementing logistics forecasting significantly reduces operational costs and improves service delivery, making it a vital component for any business looking to maintain a competitive edge.
Precise forecasting ensures that companies avoid the common pitfalls of overstocking or under stocking, which can lead to tied-up capital or lost sales.
We’ve written this guide to help fellow supply chain planners and professionals understand the intricacies of logistics forecasting and offer insights into its benefits, challenges, and best practices.
Stick with this guide to get the knowledge that drives your operations toward success.
What is Logistics Forecasting?
Logistics forecasting is the process of predicting future logistics requirements to manage resources effectively within the supply chain.
It encompasses various aspects such as demand forecasting, supply planning, inventory levels, transportation needs, and warehouse capacity.
Effective logistics forecasting involves a systematic approach that begins with the collection of relevant data from various sources, including historical performance, market trends, and external factors that could impact supply and demand.
This data is then analyzed using statistical methods and forecasting models to predict future logistics needs.
The accuracy of these predictions is crucial for planning inventory levels, workforce requirements, warehouse space, and transportation logistics, which in turn helps minimize costs and improve service levels.
Logistics forecasting is not just about predicting the future but also about continuously refining these predictions. As real-time data comes in and market conditions change, the forecasts need to be adjusted accordingly.
This dynamic process requires robust technology support, such as advanced analytics tools and software systems that can handle large datasets and complex calculations.
What are the Benefits of Logistics Forecasting?
The benefits of logistics forecasting are enhanced efficiency and effectiveness of supply chain operations, reduced costs, improved service delivery, and optimized inventory levels.
This strategic approach not only streamlines operations but also supports better decision-making processes, ensuring that companies are better prepared to meet market demands and customer expectations.
Further expanding on these benefits, logistics forecasting facilitates improved inventory management. With precise forecasts, companies can maintain optimal inventory levels, avoiding both excess stock and stockouts.
This balance is crucial for minimizing holding costs and maximizing the availability of products for timely customer delivery.
Additionally, effective forecasting helps in planning the workforce and warehouse space more efficiently, which can lead to significant cost savings and enhanced operational agility.
Moreover, logistics forecasting enhances customer satisfaction by ensuring that products are available when and where they are needed. This reliability can help build trust and loyalty among customers, which is vital in today’s competitive market.
Reduced delays and improved accuracy in order fulfillment help companies retain existing customers and attract new ones.
Thanks to accurate forecasts, a company’s ability to quickly adapt to changes in demand positions it as a reliable partner in the eyes of its clients, thereby enhancing its market reputation and competitive edge.
How does Logistics Forecasting Work?
Logistics forecasting works by systematically predicting future logistics needs through a multi-step process that involves data collection, analysis, and implementation.
This process is crucial for aligning logistics operations with business objectives and ensuring resources are appropriately allocated to meet future demands efficiently.
The accuracy of logistics forecasting significantly impacts a company’s ability to manage inventory, optimize resource utilization, and maintain competitive service levels.
Data Collection
The first step in logistics forecasting is data collection, where historical data on sales, inventory levels, market trends, and other relevant factors are gathered. This data serves as the foundation for all subsequent analysis and decision-making processes.
Advanced data collection methods, including real-time data tracking and integration of IoT devices, can enhance the accuracy and timeliness of the information gathered, providing a more reliable basis for forecasts.
Data Analysis
Following data collection, the next step is analysis, where statistical tools and forecasting models are employed to interpret the collected data and generate predictive insights.
This stage often utilizes various statistical methods such as time series analysis, regression models, and machine learning techniques to identify patterns and predict future logistics requirements.
The insights gained from this analysis are then used to make informed decisions about inventory management, workforce planning, and other logistical aspects, ultimately leading to more efficient and cost-effective operations.
What are Logistics Forecasting Challenges?
Logistics forecasting challenges are data quality, external factors, and the complexity of integrating forecasting across different supply chain components.
These challenges can impede the accuracy and reliability of predicting future logistics needs. Addressing demand forecasting challenges is crucial for maintaining efficient operations and meeting customer demands.
Quality and Availability of Data
Only accurate, complete, and updated data can lead to accurate forecasts, which can cause overstocking or stockouts. Ensuring data integrity involves not only collecting sufficient data but also regularly updating and verifying it to reflect current trends and patterns.
External Factors
The unpredictability of external factors such as economic fluctuations, political instability, or natural disasters can disrupt logistics operations and render forecasts less accurate.
These factors are often beyond the control of supply chain managers and require adaptive strategies to mitigate their impact.
Integration of forecasting efforts
Another challenge is the integration of forecasting efforts across the various components of the supply chain. Each element, from procurement and production to distribution and sales, operates with its own set of data and goals.
Aligning these disparate elements to create a cohesive forecasting model is complex but essential for achieving a unified supply chain strategy.
Strategies to overcome these challenges include improving data collection processes, employing advanced predictive analytics tools, and fostering collaboration across all parts of the supply chain to ensure a more synchronized and responsive forecasting system.
Logistics Forecasting Methods
Logistics forecasting employs various methods, from traditional techniques like time series analysis and moving averages to more advanced methods involving machine learning and AI in Supply Chains and Logistics.
The choice of method depends on the specific needs of the business, the nature of the data available, and the complexity of the logistics operations.
Traditional Forecasting Methods
Traditional demand forecasting methods, such as time series analysis, involve using historical data to predict future trends.
This method assumes that patterns observed in the past will continue, making it suitable for stable environments where changes are gradual and predictable.
Moving Averages
Moving averages are another common technique that smooths out short-term fluctuations and highlights longer-term trends in data.
These methods are beneficial for routine decision-making and operational planning in logistics.
Advanced AI Forecasting Methods
On the more advanced end, machine learning and artificial intelligence (AI) are increasingly being integrated into logistics forecasting.
These technologies can handle large volumes of data and can identify complex patterns that are not apparent to human analysts.
Machine learning models, for instance, can adjust to new data in real time, improving their accuracy over time as more data becomes available.
This makes them highly effective for dynamic environments where conditions change rapidly, such as in demand forecasting for highly seasonal products or in regions with volatile markets.
Simulation Methods
Simulation methods are also used in logistics forecasting to model and assess the potential outcomes of different logistics scenarios.
This approach allows companies to test the effects of various strategies under simulated conditions before implementing them in the real world.
Simulation can be particularly valuable in logistics for optimizing inventory levels, distribution routes, and resource allocation, thereby reducing costs and improving service levels.
These advanced forecasting methods provide logistics managers with powerful tools to make more informed, data-driven decisions, enhancing the efficiency and responsiveness of supply chain operations.
How to Forecast Logistics?
To forecast logistics effectively, businesses must systematically predict future logistics needs through a multi-step process that involves data collection, buyer-trend analysis, demand expectation analysis, and intuitive planning.
For many companies, the road to digital transformation and the implementation of AI in supply chain and logistics is not easy.
Making a shift from manual tasks to automation using modern software and tools can seem challenging and even overwhelming.
Most organizations need to realize, however, that they are creating an even more significant challenge for themselves by not introducing some of this cutting-edge technology into their operations.
Here are some of the proven ways to ensure your logistics demand forecasting is a success and accurate:
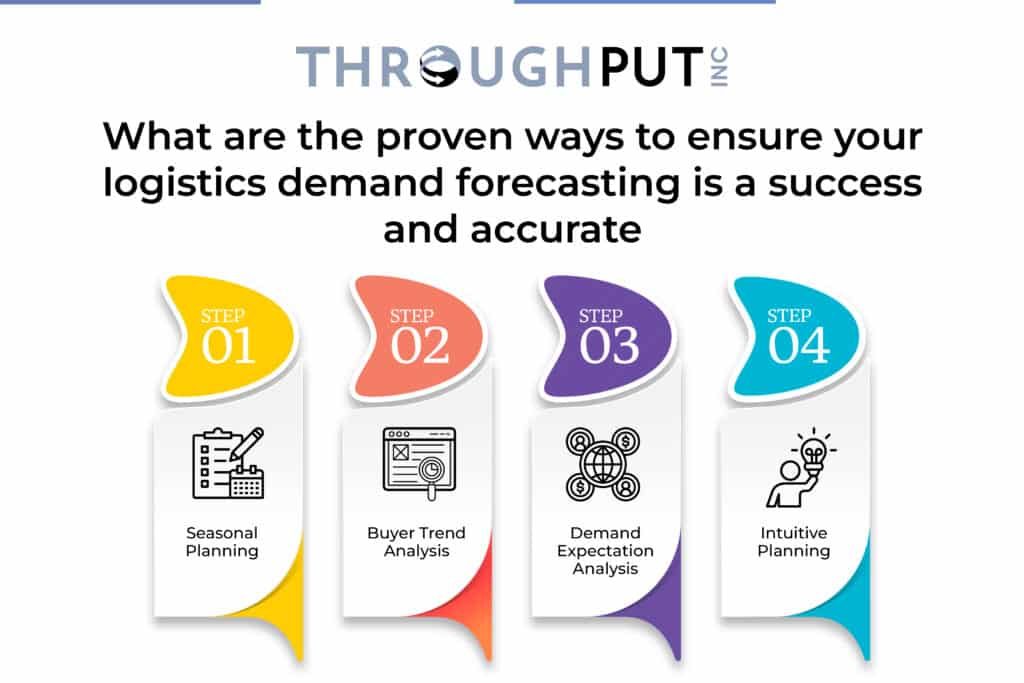
Seasonal Planning
Seasonal demand is a trend that tends to stay consistent from year to year. Logistics demand that depends on seasonal products can ensure planning for fluctuating seasonal demand shifts. This can create an accurate and specific forecast to ensure distribution on time before that season starts.
Buyer Trend Analysis
Market demand can vary depending on what consumers need at that point in time, and this happens without prior warning. Buyer trends are difficult to track and to meet buyers’ wavering demands, your logistics function needs to be flexible enough to cater to buyer-centric supply needs.
Being aware of these buyer trends can prevent manufacturers from having static inventory and keep logistics operating costs to a minimum. Fortunately, logistics makes reaching the appropriate level of inventory possible.
Demand Expectation Analysis
Demand forecasting is needed since trends fluctuate every month.
Logistics should be prepared to cater to exceptions in demand shifts, and having an effective demand exception management strategy can help manufacturers react when an expected change in demand doesn’t occur or vice versa.
Intuitive Planning
Intuition often works well in forecasting based on experience and market knowledge. Many experienced logistics and seasoned supply chain professionals can find potential shifts in consumer demand just by feeling them out.
This is called intuitive planning. Also, intuitive planning works as it can consider several factors that your SCMS can’t track. Despite not being completely technical, it can prove critical in specific industries and market conditions.
What is AI Forecasting in Logistics
AI forecasting in logistics is the application of artificial intelligence technologies to predict future logistics needs, such as demand, inventory levels, and transportation requirements.
AI algorithms are capable of processing vast amounts of data, recognizing complex patterns, and learning from historical trends to make predictions about future logistics scenarios.
When logistics operations are backed with AI, organizations benefit from enhanced forecast accuracy, improved decision-making, and increased efficiency.
The integration of AI into logistics forecasting involves the use of various techniques, including machine learning models, neural networks, and deep learning.
These AI methods can analyze data from multiple sources, including past shipment records, weather patterns, market trends, and socio-economic indicators, to provide a comprehensive view of potential logistics challenges and opportunities.
For instance, neural networks can be trained on historical data to identify the likelihood of disruptions in supply chains and suggest proactive measures to mitigate risks.
Furthermore, AI forecasting tools can continuously improve their predictive capabilities over time as they are exposed to new data. This aspect of AI, known as machine learning, allows logistics systems to become more accurate and reliable with each forecast.
The ability to adapt to changing conditions and learn from outcomes makes AI an invaluable asset in logistics planning.
As a result, companies can optimize their inventory levels, reduce waste, and respond more effectively to customer demands, ultimately leading to a more agile and responsive logistics operation.
AI forecasting is not just a futuristic concept but a practical tool that is reshaping the logistics industry today.
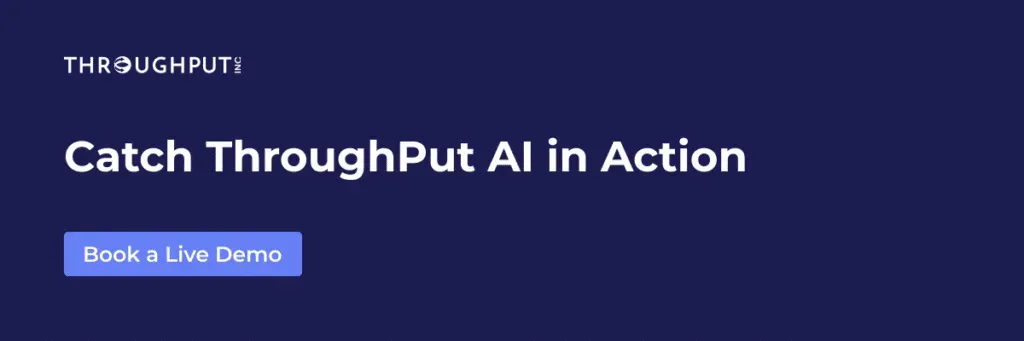