Demand Forecasting in Retail: Challenges, Solutions, and Future Trends
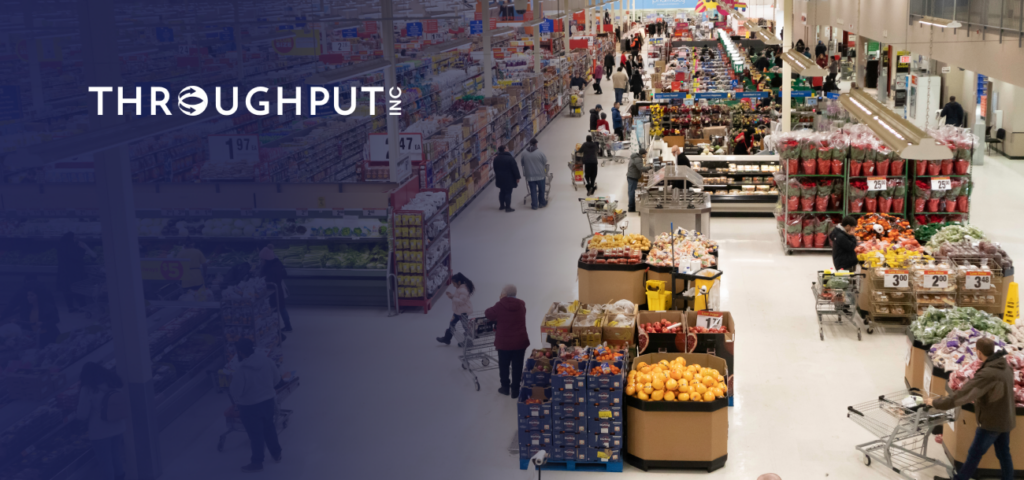
The evidence is undeniable.
Today, the $11.95 trillion global grocery retail industry finds itself at a crossroads.
Facing declining growth and profits, this sector is also grappling with soaring operational costs, dwindling productivity, and fierce competition in pricing.
Retailers are caught between the dilemma of empty store shelves and overstocked warehouses—a balancing act that demands constant attention.
In recent years, we’ve seen market dynamics shift dramatically as consumer behaviors—where they shop, what they buy, and how much they buy—have evolved rapidly and significantly.
It’s evident that food retailers particularly must rise to the occasion, thinking strategically and reacting with agility to these ever-changing market conditions, both now and in the future.
The outlook becomes even grimmer when considering the current methods of demand planning and forecasting in retail.
Most retailers’ strategies are far from a sophisticated, data-driven approach.
Repeatedly, the challenges in demand planning are ‘solved’ by referring to outdated spreadsheets that reflect static sales figures, which are then inaccurately projected onto this year’s sales performance for specific products.
It’s time for a reset, to replay and recover.
Let’s pause and digest what the data reveals:
- Commercial entities in the business world today lose almost 3% of their profits every year due to out-of-stock conditions.
- 30-40% of stockouts happen due to erroneous layout of goods in the retail sector, while 60-70% are for late deliveries by suppliers.
- In 2022, US and Canadian retailers lost sales opportunities worth almost $349 billion due to inventory distortion (total cost of overstocks and out-of-stocks).
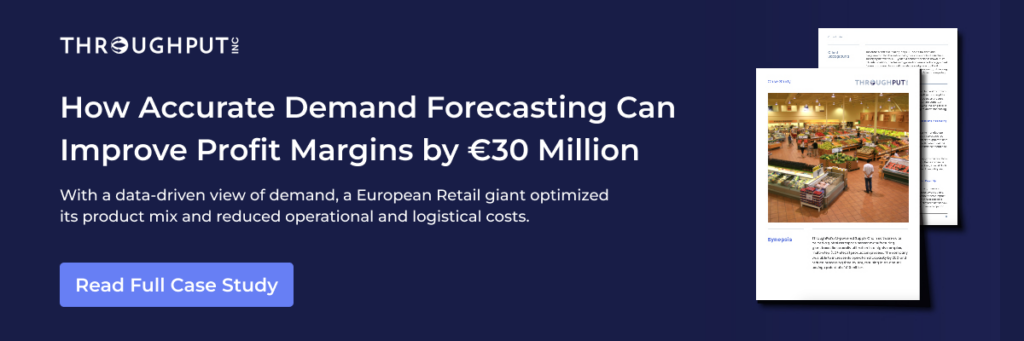
Is AI-based Demand Planning & Forecasting in the Retail Industry the Answer?
AI-based demand planning and forecasting is indeed a powerful solution for the retail industry, particularly in responding to rapid changes and unforeseen challenges.
The example of Coop, the Swiss retail giant, vividly illustrates this point. When the COVID-19 pandemic struck Europe in February 2020, Coop had to quickly deviate from its standard crisis response plans due to the unprecedented scale of the emergency.
The rapid adaptation involved forming crisis teams with broad decision-making powers, enabling them to respond swiftly and effectively without the usual bureaucratic delays.
This shift from relying on instinct to implementing AI-driven data and systems for decision-making proved crucial. It allowed Coop to accurately forecast demand and efficiently manage distribution, ensuring the availability of essential items across all stores during the pandemic.
This strategic pivot not only met customer needs effectively but also significantly boosted Coop’s market share during the crisis, demonstrating the effectiveness of AI in enhancing demand planning and forecasting in retail.
How Demand Sensing Fills the Gaps of Traditional Forecasting Approaches in the Retail Industry?
Most food retailers rely on traditional forecasting techniques that use a time-series methodology to create a forecast based on prior sales history.
But this method has a serious flaw.
While most often past sales figures are sufficient for predicting mid and long-term future plans, they usually don’t reflect the same accuracy for short-term planning.
And, incidents from across the world have shown us how important short-term plans are as market dynamics fluctuate by the day or the week.
This is where the promise of Demand Sensing steps in with a premise that – if the past is the best predictor of the long-term future, then the very recent past is the most accurate predictor of the very near future.
So, while there are a lot of demand planning theories that are based on weighted averages and other traditional methods, which might appear to be comfortable and accessible, these can only yield predictions based on historic patterns.
They lack critical raw, online and external data facts that influence the real-time view of demand and factors that affect it.
Food retailers can plan and respond to short-term changes in demand using Demand Sensing for accurate demand planning, as it is sensitive to sudden, immediate and real-time demand fluctuations that most traditional approaches fail to respond to.
In short, Demand Sensing focuses on overcoming supply chain slack by relentlessly minimizing the time lag between events and the response to those events.
By reducing the total time elapsed from the emergence of a statistically meaningful mix of demand signals, food retailers can smartly respond to those signals.
The food retail industry has always faced a massive challenge in matching supply to demand because of the vast variety of products and the fluctuating sales trends in the market.
Accurate Demand Sensing can go a long way in reducing the difficulties of traditional demand forecasting in retail.
- The Dilemma of Stockout Vs Overstocks
Poor forecasts can lead to unexpected stockouts which eventually results in lost sales opportunities.
Since customers expect stores to have a consistent stock and availability of essential supplies, stockouts can reduce customer footfall and loss to the competition.
Similarly, overstocking can engage valuable capital in inventory, leading to wastage, markdowns, etc., to get rid of old stocks.
Food Retailers could find it difficult to strike the right balance for increasing profit margins.
- The Plague of Legacy Forecasting Systems
Legacy systems for demand forecasting in retail are usually the trigger of the problem.
Most of these systems are rigid and don’t account for real-time event changes, weather fluctuations, and local variables.
They also lack holistic visibility into the several factors that drive consumer purchasing decisions and thus create challenges for retailers to immediately respond to their customers’ day-to-day needs.
Due to this food retailers can suffer with excess or limited inventory, lost sales opportunities and unnecessary product waste that needs to be discarded.
- The Vagueness of New Product Introductions
Forecasting accurately for new product introductions is now extremely critical as the product life-cycle shortens and assortment turnover grows. For the food retail industry, where new product introductions are very common and continuously restocked, this forms a significant bulk of the sales.
So, if there is an error in the demand forecasts for product introductions, you may not accurately create overall business forecasts.
- The Fluctuating Variance in Sales Volume
Retail managers have to deal with continuous changes in sales volumes especially in advanced retail environments.
Demand forecasting in retail needs to account for these fluctuations using a combination of statistical forecasting complemented with human insight.
Many times, sales variances can also occur due to a larger market condition and this is where forecasting methods need to be sensitive to short-term changes and step up to infer a causal link from generic events.
Demand Response Plan in Retail With Intelligent Demand Sensing
Demand Sensing can be extensively used across several layers in the food retail industry.
By adopting Artificial Intelligence and Machine Learning-driven rapid demand sensing, food retailers and supply chain managers can easily mitigate several risks associated with it, as described below.
These techniques are specially built to incorporate key information quickly enough to act upon agile supply chains in real time.
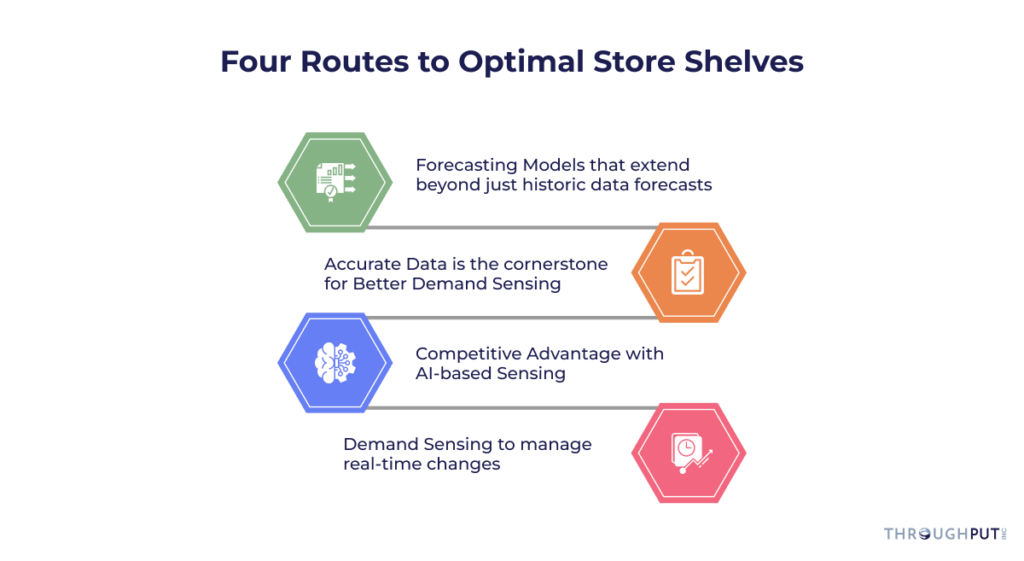
1. Forecasting models that extend beyond just historic data forecasts
AI-driven demand sensing models that can self-learn from other items and stores to generate accurate forecasts despite having limited data, would work the best in the long run.
These algorithms will be able to extract detailed information from other parallel marketing events to finally predict how a new product or service will react to variations in prices, demand trends, etc.
FOOD-RETAILER TIP: Look beyond just historic data to be able to prepare for future demand fluctuations. This will ensure you can evaluate and respond to any changes across multiple locations and decide if these fluctuations represent a whole or a single isolated incident
2. Accurate data is the cornerstone for better Demand Sensing
To ensure a higher forecasting accuracy, you need to move beyond just looking at the methods for annual or quarterly demand planning.
The data that matters the most depends on the day-to-day activities being done to fulfill daily orders and purchases at the store.
If these daily numbers are off, your forecasting accuracy can get greatly skewed and this can impact the final performance of your overall supply value chain as well.
FOOD-RETAILER TIP: A demand forecasting solution that can be integrated across all your sales channels can ensure more than 100% forecasting accuracy.
Just make sure these models pick up the granular data across these sales channels at every level including seasonal fluctuations, holidays, and customer trends, and then correlate this data and map it accordingly at regular intervals.
3. Competitive advantage with AI-based sensing
Europe’s largest retail food chain aimed at implementing a data-driven review of fulfillment and shipping practices for their extensive food warehouses to optimize replenishment and allocation down to individual SKUs.
Due to limited insights into the existing siloed and legacy data systems, the food retailer wanted an accurate flow analysis between warehouses and to optimize the process of shipment handling and transport processes to drive higher overall profit margins.
Using ThroughPut’s AI-powered Supply Chain Intelligence solution, the retailer was able to create $20 Million of annual savings in their supply chain from one project alone, resulting in a 10% reduction in trucking costs, a 1% increase in top-line revenue, and a 2X improvement in inventory turns.
Read: AI-powered demand sensing: 7 Strategies To Increase Sales Performance.
FOOD-RETAILER TIP: An AI-driven Demand Sensing solution results in a more accurate and automated pull-based replenishment, creating a highly profitable push-based replenishment.
These algorithms are constantly learning and can provide real-time views to respond accurately to sudden demand fluctuations.
4. Demand Sensing to manage real-time changes
Customer changes can be hard to keep up with, especially if you are forecasting way out into the future. To account for near-term changes, a more real-time approach is needed.
FOOD-RETAILER TIP: Using a Demand Sensing solution can help reduce forecasting errors by 30%-40% as it enables retailers to constantly address sharp changes in customer needs and further helps build a data-driven supply chain.
What Are the Benefits of Demand Sensing in Retail?
Demand Sensing goes one step ahead with a host of more granular benefits.
- Ensures forecast accuracy from the traditional 60% to over 80% right at the SKU/ shelf/ location level.
- Synchronizes between demand planning and execution while helping the user to get a deeper understanding of varying customer behavior by evaluating the multiple costs across multiple outcomes.
- Balances inventory requirements, reduces wastage and cuts down logistics and transportation costs.
- Facilitates accurate, timely and informed decision-making about inventory, pricing, replenishment, and assortment at a much more granular level.
- Streamlines upstream planning by extracting relevant data right from the Point-of-Sale (PoS) to improve forecasting accuracy without dependency on distributors or suppliers.
- Boosts production-planning efficiency by providing regular inputs to supply operations which helps tweak production plans by expediting orders.
- Fills in and syncs up the inbound and outbound gaps across warehouses to reflect a unified picture of global demand.
- Optimizes inventory and balances the supply planning efficacy by accounting for available inventory across regional warehouses, complemented with predicted customer demand, by location.
- Forecasts seasonal demand for better supply chain responsiveness to help food retailers’ production and inventory to avoid obsolescence.
- Manages new product introductions with the right-size inventory to tackle demand outstrips and maintain optimum inventory levels of new and older product versions based on customer demand.
- Improves marketing promotion evaluation and performance to help planners understand the factors influencing promotional uplifts and better plan for future promotions.
Accurate Demand Forecasting in Retail With ThroughPut AI’s Demand Sensing Module
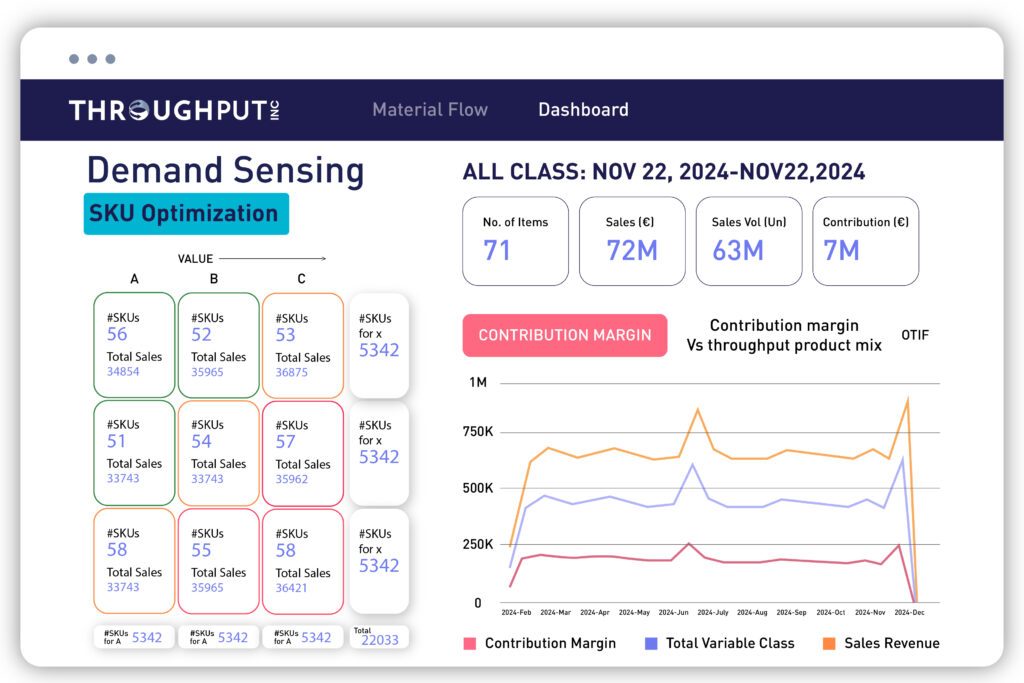
The ThroughPut Demand Sensing Module is a data-driven, go-to planning and forecasting tool that accurately analyzes and correlates demand insights and responds to fluctuating margins and inventory needs.
“Demand Sensing is the first step of putting your end-to-end supply chain on autopilot. […]this module will ensure that customers never miss out on customer demand again due to operational constraints.” Ali Raza, Founder & CEO, ThroughPut Inc.
Get Organizations Closer to Near Future Demand Patterns
ThroughPut’s Demand Sensing Module emulates a retail supply chain planning software powered by AI that helps you to easily predict near-future demand patterns and streamline the flow of materials, processes, output, and free cash flow across your integrated supply chain.
- Rapidly analyze & correlate demand-based insights in an easily digestible format.
- Improve the overall product mix and service availability and enhance revenue margins.
- Accurately forecast and respond to fluctuating margins and inventory needs.
- Identify & expose patterns across past events and quickly predict demand trends.
Empower Supply Chain Demand Planners With Business-critical Intelligence
Demand planning has now become a critical function that forms the foundation of business-critical intelligence for the entire organization.
This means it would need equal participation by various stakeholders as it impacts profit margins, revenue streams, capital flow, and resource allocation.
The Demand Sensing Module addresses this collaborative need through its salient features:
1. Connect the Dots Between Past, Present, and Future
ThroughPut’s Demand Sensing Module uses historical product/service sales data to draw up a time-series pattern and analyze the short-term trends.
It also takes into account external events, holidays, and promotions to accurately identify, predict, and measure future demand patterns to enhance product, and service availability and boost revenue margins.
Business Outcome: Accurate demand sensing eliminates time-consuming manual efforts and streamlines operational priorities.
2. Confidently Work with a 360-Degree View of Your Demand Plans
Demand planners need a holistic approach using data right from a granular level to a panoramic view to make informed product-mix decisions.
With the Demand Sensing Module, you have this data at your fingertips.
Business Outcome: A multi-dimensional view drives accurate data-driven decisions from the ground up, enabling better productivity and collaboration.
3. Group Sellable Products Based on Logical Segmentation
An ABC/XYZ Segmentation of sellable products or services based on grouping, geo-location, and product mix helps you to quickly optimize your product mix by better understanding top and low-performing SKUs.
Business Outcome: A ready-to-plug-and-play segment-wise analysis of your product mix across locations, product groups, and SKUs.
4. Extract Demand Summary and Augmentation Views Across Variables
The Demand Sensing Module provides a cross-sectional summary view including Throughput, Lost Sales, and OTIF/demand trends. You can also load and test additional regressors against demand across holidays, promotion events, weather changes, etc.
Business Outcome: Deep-dive across variables that could affect demand forecasts while taking into account possibilities of variations based on market trends.
5. Track Shifts in Market Sentiments and Customer Behavior with Accuracy
The Demand Sensing Module helps generate forecast plans, and recommend inventory levels and operations plans that match the market sentiments at a given point in time. It also keeps the operations costs under control.
Business Outcome: Make sure your products are optimally stocked irrespective of erratic shifts in market behaviors and patterns.
6. Eliminate Operational Waste by Calculating Procurement vs. Wastage
The Demand Sensing Module considers external influences, including economic, geographical, and climatic consequences.
You can, therefore, accurately quantify your input vs. output without incurring wastage.
Business Outcome: Understanding how to realign demand sensing in the supply chain to avoid unnecessary waste can give you a competitive edge.
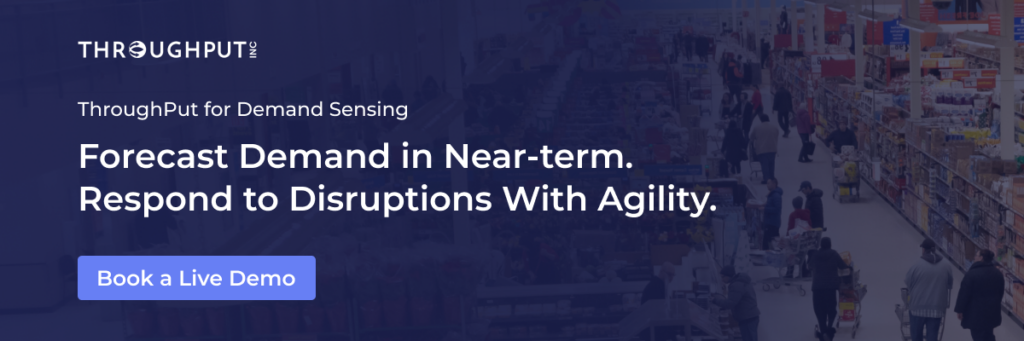
What Are the Future Trends in Demand Sensing & Demand Forecasting for Retail?
As we look towards the future of demand sensing and demand forecasting in retail, several trends stand poised to reshape the industry.
The integration of Artificial Intelligence (AI) and machine learning is revolutionizing the landscape, enabling precise, real-time predictions based on diverse data sources and economic indicators.
This shift not only improves inventory management but also enhances customer satisfaction by reducing stockouts and excess inventory.
Furthermore, the use of big data analytics allows retailers to dive deeper into understanding consumer behaviors and preferences, enhancing predictive capabilities.
The Internet of Things (IoT) is another transformative technology, with RFID tags, connected sensors, and smart shelves providing continuous data on inventory levels and product movement, thus enabling informed decision-making and optimized supply chain operations.
Omni-channel integration is becoming essential as consumers increasingly shop across multiple platforms. This integration ensures that inventory aligns perfectly with consumer demand across all channels, improving the shopping experience and opening doors to new sales opportunities.
Additionally, the rise of collaborative forecasting promotes holistic approaches by sharing demand data across the supply chain, improving coordination and alignment among partners, vendors, and suppliers.
Lastly, sustainability considerations are increasingly influencing demand sensing. As consumer awareness of environmental impacts grows, retailers are incorporating sustainability into their forecasting models, tracking demand for eco-friendly products and striving to reduce waste.
These advancements signify a dynamic shift towards more integrated, intelligent, and responsive retail operations, promising not only to meet consumer needs more effectively but also to drive the industry forward in exciting new directions.