Demand Sensing Benefits for Supply Chains
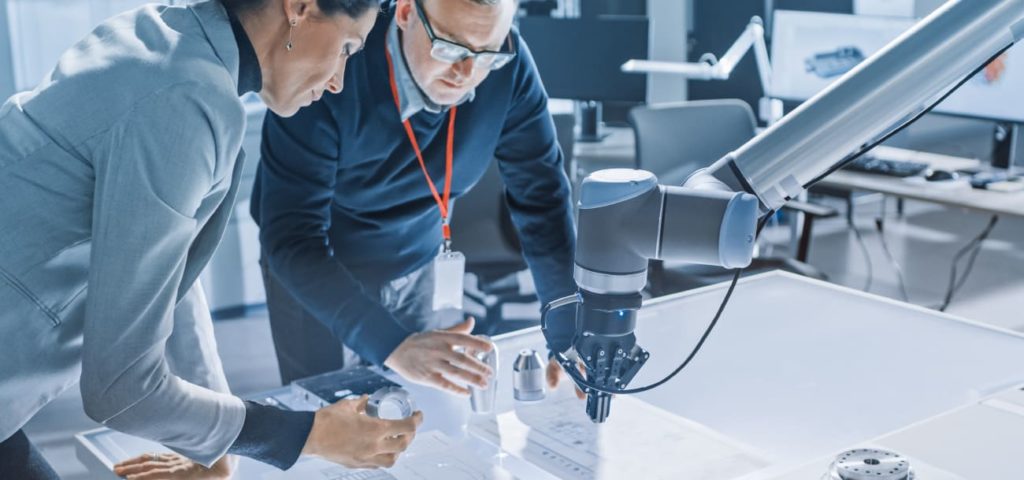
It’s hard to imagine, but there was a time when customer demand didn’t drive business and supply chains. Customer preferences hardly affected supply chain choices, production schedules, or inventory levels. And surprisingly, this wasn’t eons ago – it was just around twenty years back!
Today, the ability to accurately forecast customer preferences and demand is at the heart of any supply chain’s success. The ones that manage to navigate disruptions and fluctuating customer demand have an undeniable upper hand over the rest.
But anything close to a cent percent accurate demand forecasting is a distant dream for all demand planners.
With the pandemic and the wave of uncertainties, getting the right products in the right quantity at the right place has only gotten more challenging. In the face of failing traditional forecasting techniques, you are most likely looking for a more reliable solution for forecasting demand in this new norm.
And that’s where demand sensing comes in.
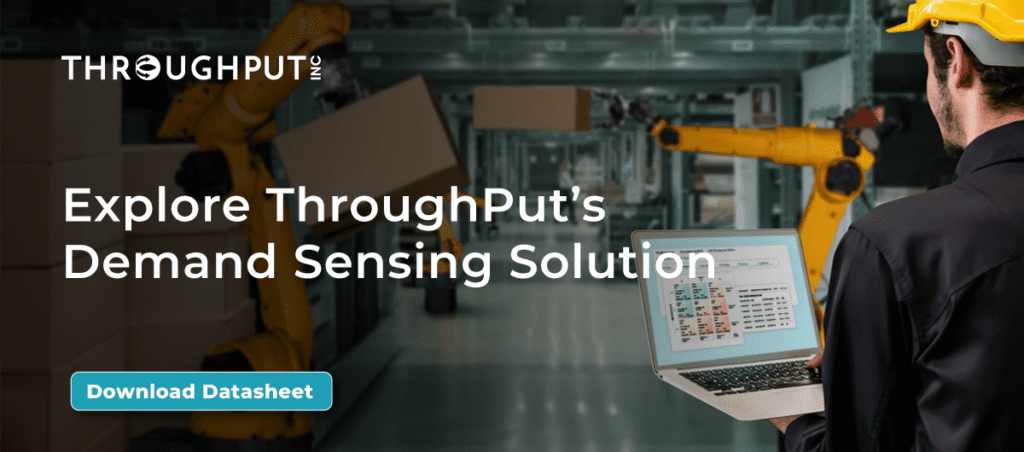
So in this article, we’ll delve into what demand sensing is, how it diverges from traditional forecasting and the mechanics behind the demand sensing process. Let’s dive in!
What Is Demand Sensing in the Supply Chain?
Demand sensing is an advanced forecasting technique in supply chain management that turbocharges demand predictions’ precision and responsiveness.
The technique leverages advanced analytics and machine learning algorithms to generate short-term and mid-term forecasts based on the current realities of the supply chain.
Demand sensing taps into real-time data like point-of-sale figures and market intelligence to capture current customer demand signals. These signals then guide adjustments in supply chain plans.
Demand sensing is a lifesaver, especially in industries prone to fast-changing market dynamics, seasonal shifts and customer preferences.
With proper demand sensing in place, companies can adjust their demand plans and inventory levels with more accurate demand forecasting, enhanced customer satisfaction, reduced inventory costs and improved agility.
What Are Demand Sensing Techniques?
Common demand sensing techniques include:
- Point-of-sale (POS) data analysis that analyzes real-time sales data from POS terminals to capture demand signals and adjusts inventory levels accordingly.
- Social media monitoring tracks social media activity and sentiment to identify trends and changes in consumer behavior that may impact demand.
- Weather data analysis incorporates weather forecasts and historical weather data to predict the impact of weather events on demand patterns.
- Economic indicators such as GDP growth, unemployment rates and consumer confidence predict changes in demand.
- Machine learning algorithms analyze large volumes of data and identify patterns, trends, and relationships that can help predict demand.
What are the Benefits of Demand Sensing in Supply Chain Management?
Demand sensing offers several advantages over traditional forecasting, particularly in dynamic and rapidly changing business environments. Here are some key benefits of demand sensing:
- Demand sensing relies on real-time data sources, providing up-to-the-minute insights into current customer demand.
- Since demand sensing relies on real-time data, it also excels at capturing short-term fluctuations and changes in customer behavior.
- Demand sensing enables rapid production, distribution, and inventory adjustments based on real-time demand signals.
- Demand sensing considers external factors like promotions, events, and market shifts that can impact demand. The external factor analysis enhances the accuracy of predictions, especially in unpredictable scenarios.
- Real-time insights enable various departments to align their efforts, improving collaboration.
- Accurate demand sensing helps maintain product availability, ensuring that customers find what they need when they need it, which leads to improved customer satisfaction and loyalty.
- By accurately predicting demand and minimizing stockouts, companies reduce carrying costs associated with excess inventory and optimize their supply chain operations.
- Demand sensing relies on data analysis and advanced algorithms, reducing the impact of human biases and enhancing decision-making quality.
- In times of uncertainty, such as during disruptions or crises, demand sensing can offer better risk management through insights into evolving market dynamics.
The ability to incorporate real-time data, respond quickly to changes, and adapt to dynamic market conditions makes demand sensing a powerful tool for accurate and agile demand forecasting metods.
What are the Differences Between Demand Sensing, Demand Forecasting and Demand Planning?
Demand planning, demand sensing, and demand forecasting are interrelated but distinct concepts within supply chain management. Let’s compare them to understand the differences.
Demand Sensing vs Demand Forecasting
If you’ve noticed traditional demand forecasting tools stumbling lately, they heavily lean on historical data and expect past trends to continue.
Traditional forecasting doesn’t note abrupt disruptions and swings that have become more common, especially since the pandemic hit.
This is where demand sensing steps in, plugging the gaps left by traditional forecasting. Real-time insights provided by demand sensing enable businesses to adapt to market shifts quickly.
Traditional forecasting guides strategic mid- and long-term planning, and demand sensing empowers short-term decisions, making supply chains more agile and customer-focused.
Point of comparison | Modern Demand Sensing | Traditional Demand Forecasting |
Data Source and Timing | Utilizes real-time data sources such as point-of-sale data, social media trends, and market intelligence to capture up-to-the-minute customer demand signals. | Relies on historical data and statistical models to make predictions based on past patterns. |
Time Horizon | Focuses on short-term and near-term forecasts, usually covering weeks to a few months ahead. | Primarily addresses medium- to long-term forecasts, often covering several months to years. |
Granularity and Detail | Provides a more detailed and granular view of demand by capturing fluctuations, sudden shifts, and external influences. | Offers a broader overview of trends and averages but may not capture short-term variations effectively. |
Flexibility and Responsiveness | Allows for quick adjustments in response to sudden market changes, promotions, events, and shifts in customer behavior. | May be less responsive to rapid changes in demand, as it relies on historical patterns that might not capture current dynamics. |
Accuracy and Precision | Aims to achieve higher accuracy in short-term predictions by incorporating real-time data and current market signals. | Can have varying accuracy levels, with greater challenges in capturing short-term variations accurately. |
Technology and Tools | Requires advanced analytics, data integration, and real-time monitoring tools to process and interpret dynamic data streams. | Often relies on statistical methods and historical data analysis, with less emphasis on real-time data integration. |
Demand Planning vs Demand Sensing
Demand planning is a multi-step process that includes forecasting or sensing demand. It aligns supply chain and business strategies to ensure that the various supply chain elements are synchronized to meet customer needs.
The scope of demand planning includes demand forecasting but goes further by integrating collaboration across business functions – sales, marketing, and finance – to meet the forecasted demand. This collaboration ensures an integrated response to the demand forecast.
As explained in the above section, demand sensing leverages real-time data and advanced analytics to detect changes in demand as they are happening. So, demand planning based on demand sensing is far more effective in managing short-term fluctuations in demand.
Bringing all three concepts together shows that:
- Demand forecasting predicts future demand and is a part of demand planning. However, it doesn’t include the demand sensing’s broader collaboration or real-time responsiveness.
- Demand sensing is all about understanding and reacting to immediate changes in demand using real-time data. It focuses on short-term forecasts and immediate stock replenishments.
- Demand planning is a more comprehensive process that includes demand forecasting or demand sensing as a subset to align the entire organization’s strategies to meet expected demand.
All concepts help effectively plan, understand, and respond to the product or service demand but play a unique and different role within the larger supply chain strategy.
Why does Demand Sensing Require AI?
Using AI for demand sensing brings a new level of accuracy, responsiveness, and insight to forecasting customer demand. AI-enabled demand sensing eliminates any possible supply chain lag by continuously reducing the time between events and the supply chain’s response to those events.
Most organizations that aim to reap the benefits of accurate demand sensing turn to AI technologies. Among other things, AI algorithms make it possible to process vast amounts of real-time data, identify complex patterns and non-linear relationships in data and continuously improve accuracy over time. AI models also quickly adapt to changes in demand patterns, even in the face of unprecedented events.
Data fuels AI-powered demand sensing. The accuracy, effectiveness, and insights derived from demand sensing heavily rely on the quality and breadth of data used. Here’s how data influences AI-powered demand sensing:
- Incorporating external factors like weather, holidays, economic indicators, and competitor activities enriches the AI model’s understanding of demand drivers.
- Machine learning training and AI algorithms learn from historical data to identify patterns, trends, and relationships influencing demand — the more comprehensive and diverse the training data, the better the algorithm’s predictive capabilities.
- The more granular the data, the more detailed the demand predictions. Data at the SKU level, geographic regions, customer segments, and even specific time frames contribute to more accurate forecasts.
- Data reflects the unique characteristics of your business and industry. AI models can be customized to consider industry-specific factors that impact demand.
- Scenario simulations paired with diverse and comprehensive data allow AI models to perform “what-if” scenarios to anticipate the impact of various market changes on demand.
- Comparing actual demand with forecasted demand creates a feedback loop. This loop helps the AI model refine its predictions over time, improving its accuracy.
What Are Some Modern Demand Sensing Technologies and Solutions?
Demand sensing technologies and solutions help businesses better understand and predict customer demand. These technologies use real-time data, advanced analytics, artificial intelligence and machine learning algorithms. Some popular demand sensing solutions include:
- ThroughPut Demand Sensing:
This is our in-house developed, AI-powered solution that taps into existing data tied to sales, purchasing, distribution, and manufacturing activities, helping businesses optimize their supply chain operations and meet customer demand in real time. It captures short-term fluctuations in demand and enables you to predict near-term demand more accurately in these uncertain times. - E2open Demand Sensing is a software that uses AI and machine learning technology to analyze real-time supply chain data and generate near-term demand predictions with better forecast accuracy than traditional approaches.
- ToolsGroup Demand Sensing Software is another demand sensing solution that helps businesses extract signals from data and react to downstream demand to better forecast accuracy, reduce inventory, and enhance customer service levels.
- o9 Solutions Demand Sensing is a platform that enables companies to identify short-term trends and market shifts to predict consumers using AI and machine learning algorithms.
- RELEX Solutions Demand Sensing leverages data and machine learning to improve accuracy and flexibility in demand forecasting. The solution is specialized for the fast-moving consumer goods (FMCG) industry.
These demand sensing solutions aim to achieve similar things – help organizations optimize their supply chain operations, enhance efficiency, reduce costs, and improve customer satisfaction.
We may be biased, but no tool provides such a significant wealth of information and actionable insights as ThroughPut AI.
So, let’s check the demand sensing process with ThroughPut AI.
What Does an AI-powered Demand Sensing Process Look Like?
At ThroughPut, we take a 7-step approach to demand sensing as follows. While it is not the only way, we’ve been enabling our customers to build successful demand-driven supply chain management practices with this methodology.
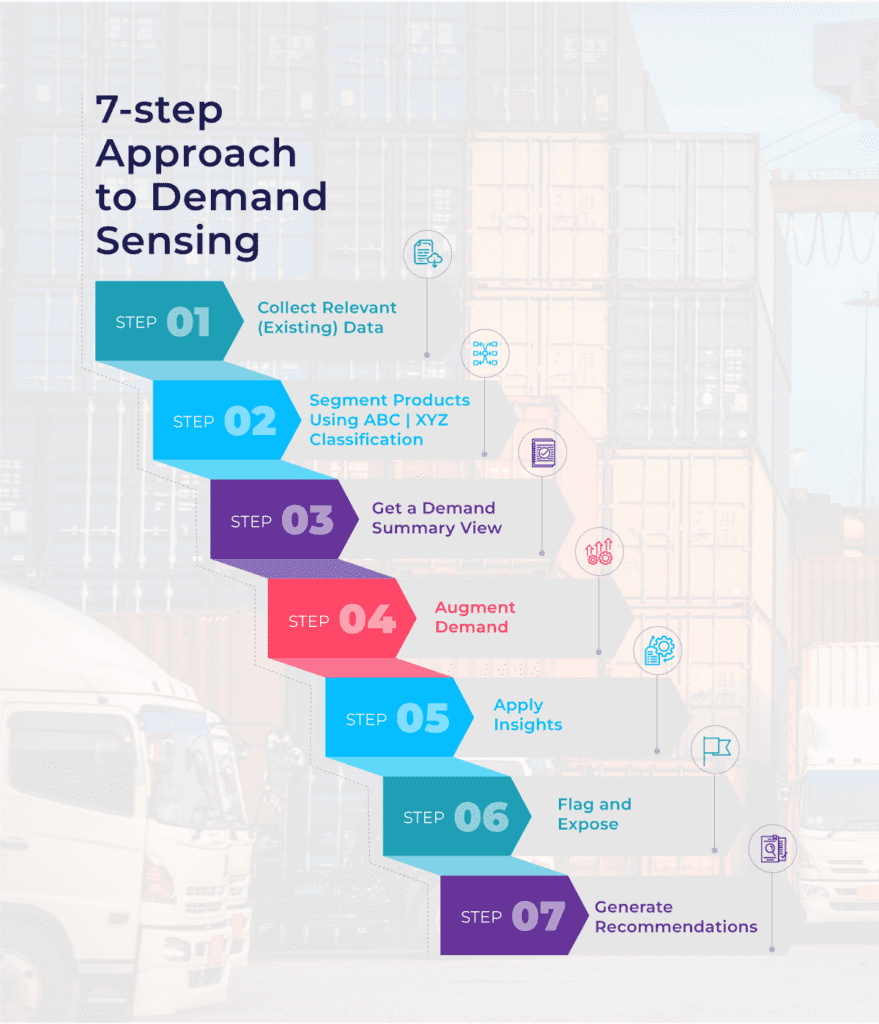
Step 1: Collect Relevant (Existing) Data
Gathering relevant data is the foundation of demand sensing. The data collection includes historical sales data, customer orders, point-of-sale (POS) data, market trends, weather information, and other external factors.
Integrating these diverse data sources creates a comprehensive dataset that forms the basis for accurate predictions.
Often, businesses believe they don’t have enough existing data to work with. That’s far from the truth. ThroughPut has been effectively working with existing data to get faster results.
Step 2: Segment Products Using ABC | XYZ Classification
The data is analyzed to categorize products based on demand predictability or reliability and their volume or consumption. The ABC classification divides products into categories based on their value or contribution to the business.
“A” items are high-value products that contribute the most to revenue, “B” items are moderately valuable, and “C” items are lower in value.
The XYZ classification categorizes products based on their demand predictability, reliability, volume, or consumption. “X” items have the most stable and predictable demand, “Y” items have moderate predictability, and “Z” items have the least predictable demand.
This level of product segmentation gives both a high-level perspective, allowing the identification of broad trends and patterns across product categories and a granular view that focuses on specific data and characteristics for each product.
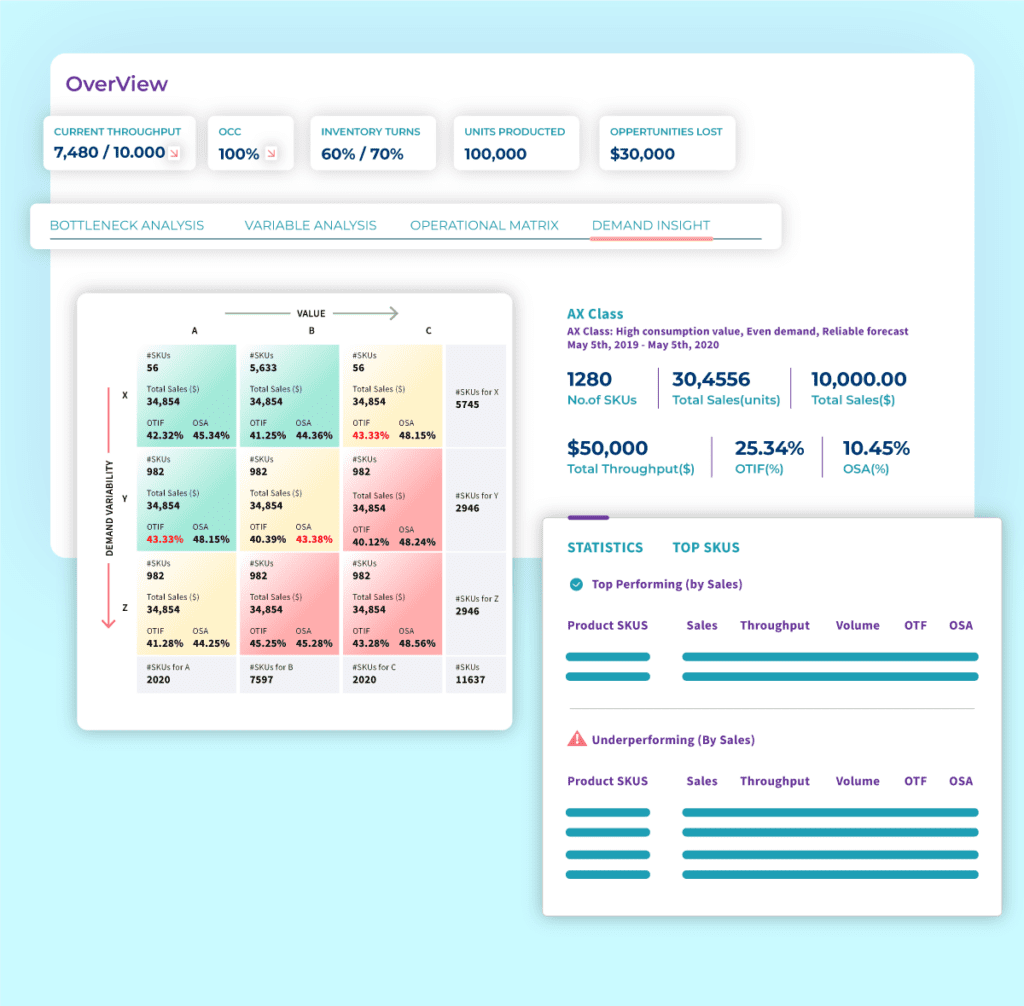
Step 3: Get a Demand Summary View
The demand summary view consolidates crucial metrics into a single snapshot, clearly understanding how effectively the supply chain is currently operating to meet customer demand for each product.
By monitoring Actual Throughput, Lost Throughput, On-time In-full (OTIF), and On Shelf Availability (OSA) performance, companies can identify areas for improvement, optimize inventory management, enhance customer satisfaction, and drive more efficient supply chain operations.
Step 4: Augment Demand
Demand augmentation involves enhancing demand forecasting accuracy by incorporating additional factors or variables, known as regressors, that may impact customer demand. These factors could include holidays, promotional events, weather conditions, economic indicators, and other external influences.
By augmenting demand forecasting models with additional regressors, companies can capture the influence of external factors that might otherwise be overlooked in traditional forecasting.
This approach enhances the accuracy of predictions, making them more reflective of real-world demand patterns and enabling better supply chain planning and decision-making.
Step 5: Apply Insights
In this step, extract insights from cyclical or seasonal trends and past regression effects on demand, and incorporate these insights into the demand forecast.
For instance, if the system identifies a consistent increase in demand during holiday seasons, use this knowledge to adjust the forecasted demand during upcoming holidays.
ThroughPut uses ELI, an advanced AI system designed to uncover hidden patterns and relationships in data at this step. It goes beyond predicting outcomes and focuses on explaining the underlying factors contributing to those outcomes.
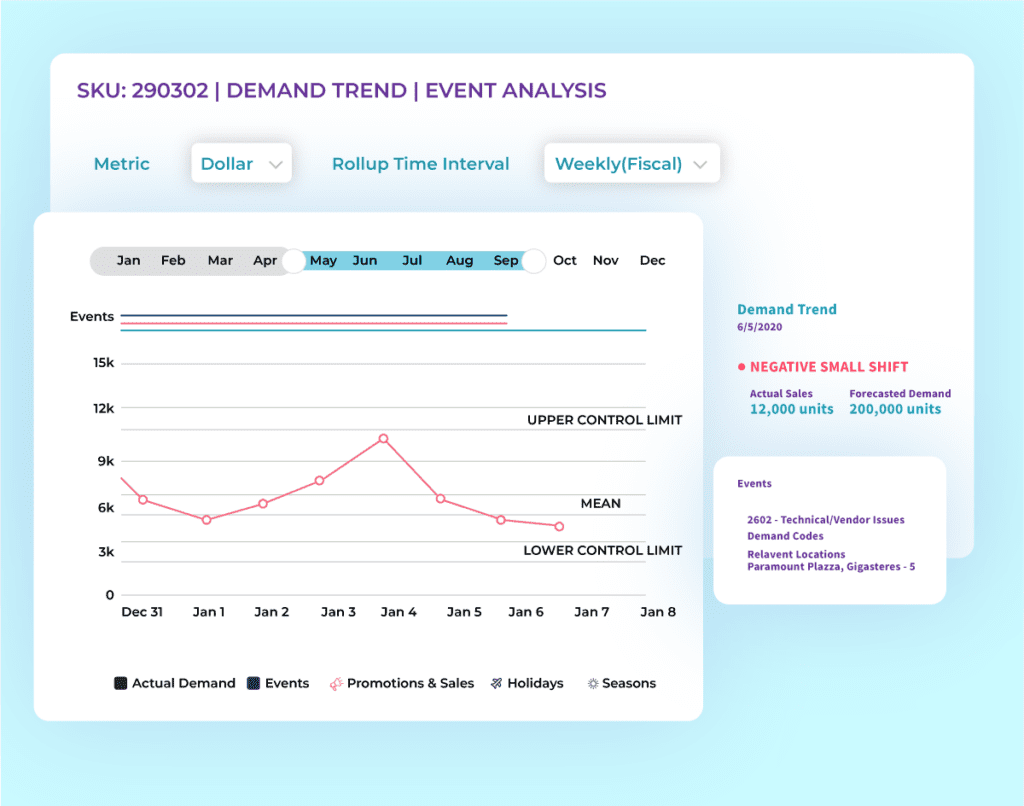
Step 6: Flag and Expose
This step entails analyzing data to recognize patterns where certain events or conditions consistently coincide with shifts in demand. When these correlations are identified, they are highlighted for further investigation and analysis.
Quantifying the strength and significance of these correlations helps understand their impact on demand. Insights derived from these relationships can inform decision-making, allowing businesses to anticipate demand fluctuations and adjust their strategies accordingly.
By incorporating these correlations into scenario simulations, companies can explore how changes in specific factors might influence future demand. Monitoring and updating these correlations ensures supply chain operations and strategies remain aligned with dynamic market conditions.
However, it’s important to note that while correlations suggest a relationship, they don’t necessarily imply causation and careful analysis is needed to draw meaningful conclusions.
Step 7: Generate Recommendations
Once the demand forecast is established, the next step is to derive recommended inventory levels and operational plans. The planning involves aligning inventory quantities with the predicted demand, ensuring that products are available when customers want them.
Optimal inventory levels mean corporations can balance avoiding stockouts and minimizing excess inventory, leading to improved service levels.
Moreover, the recommended operations plans consider the predicted demand, ensuring that production schedules, procurement strategies, and other operational activities are synchronized with market requirements.
The alignment helps optimize working capital by preventing overstocking or understocking situations. It also controls operational expenses by streamlining resource allocation and supply chain operations based on real-time and forecasted demand patterns.
The whole process enhances customer satisfaction through better product availability, supports efficient resource utilization, and fosters prudent financial management.
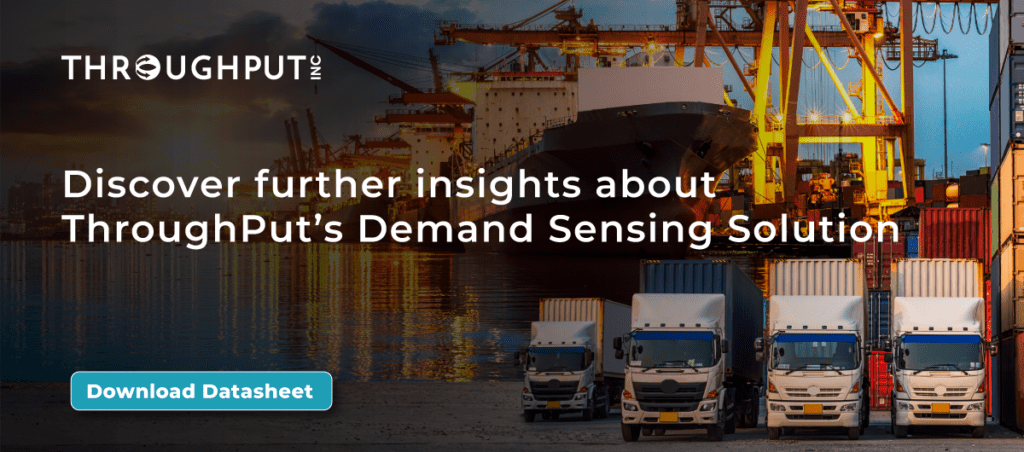
ThroughPut’s Demand Sensing Solution – Turn Insights into Action
ThroughPut’s demand-sensing solution can aid your company in understanding demand triggers and deploying demand-shaping strategies effectively.
With automated real-time data and advanced AI and ML algorithms, the system generates daily demand forecasts that adapt to evolving customer requirements and market dynamics. These insights empower companies to transform sales data into operational insights that outperform competitors.
Integrating ThroughPut’s solution into business operations is not complicated. Learn more about the benefits offered by ThroughPut’s demand-sensing module.