Traditional demand planning methods often need to catch up due to their reliance on historical data and linear forecasting models, which fail to account for the dynamic nature of modern markets.
This information gap leads to forecast inaccuracies, which result in overstock or stockouts and ultimately affect customer satisfaction and profitability.
What is Demand Planning?
Demand planning is a critical component of supply chain management that involves forecasting future customer demand to ensure that a company can meet its objectives optimally.
The primary goal of demand planning is to balance the inventory levels with the anticipated customer demand, thereby minimizing costs while maximizing service levels and profitability.
Integration into Supply Chain Management
Demand planning does not operate in isolation but is integral to broader supply chain management. It interacts with other supply chain processes such as supply planning, procurement, manufacturing, and distribution.
Effective demand planning leads to a more responsive and agile supply chain capable of adapting to market conditions and changes in customer preferences.
When demand forecasts, production schedules and inventory levels are aligned, companies can create a synchronized supply chain that not only meets customer demands efficiently but also reduces costs and enhances profitability.
This integration is crucial for maintaining a competitive edge in the market, as it allows for quick adjustments to supply chain operations in response to changing demand patterns.
What are the Problems with Traditional Demand Planning Methods?
The problems with traditional demand planning methods are the existence of data silos, inaccurate forecasts, and lack of responsiveness.
Traditional demand planning methods often need help with the efficiency and effectiveness of supply chain operations.
These challenges primarily stem from outdated practices, technological limitations, and organizational silos, which can lead to inaccurate forecasts and a lack of responsiveness to market changes.
Common Challenges and Limitations
- Data Silos
Traditional demand planning often operates in silos where information is not shared effectively across different departments or stages of the supply chain. This lack of integration leads to inconsistent data for planning and forecasting, resulting in inefficiencies and increased chances of error.
- Inaccurate Forecasts
Traditional methods frequently rely on historical data and linear forecasting models that may not account for complex market dynamics or sudden changes in demand patterns. This can lead to forecasts that do not accurately reflect current market realities, causing either overstock or stockouts.
- Lack of Responsiveness
Traditional demand planning methods are often rigid and must be more agile to respond quickly to market changes. This sluggish response can be detrimental in fast-paced industries where consumer preferences and competitive landscapes evolve rapidly.
- Bullwhip Effect
A significant issue in traditional supply chains is the bullwhip effect, where small variations in demand at the consumer level cause progressively larger fluctuations in orders and inventory upstream. Poor information flow and forecasting practices exacerbate this effect, leading to inefficiencies and increased costs throughout the supply chain.
Impact on Business Operations and Profitability
The limitations of traditional demand planning methods can have profound impacts on business operations and overall profitability:
- Increased costs due to inaccuracies in demand forecasting can lead to excessive inventory holding costs, wasted resources, and increased operational expenses. Additionally, the bullwhip effect can cause significant inefficiencies and cost escalations across the supply chain.
- Reduced customer satisfaction because of failing to meet customer demand due to stockouts or delays can lead to decreased customer satisfaction and loyalty. Conversely, overstock resulting from inaccurate forecasts can tie up capital that could be used more effectively elsewhere.
- Lost Sales and market share due to the inability to respond swiftly to market changes can result in lost sales opportunities and diminished competitive edge, as competitors who are more agile can capture market share by more effectively meeting customer demands.
- Operational inefficiencies of traditional methods that do not utilize advanced analytics or real-time data are often less efficient, leading to suboptimal production schedules, increased lead times, and a higher likelihood of errors in order fulfillment.
How can AI Improve Demand Planning?
AI improves demand planning by introducing real-time data processing, adaptive learning for demand forecasting, and integration with inventory management.
Artificial Intelligence (AI) has significantly transformed demand planning by introducing capabilities extending far beyond traditional methods’ reach.
These advancements enhance the accuracy and efficiency of demand forecasts and integrate seamlessly with modern technologies like the Internet of Things (IoT) to revolutionize inventory management.
Below, we delve into specific improvements AI brings and explore real-world examples of AI successfully enhancing demand planning outcomes.
Real-Time Data Processing
AI excels in handling and processing large volumes of data in real time. This capability allows businesses to react swiftly to changes in demand patterns as they occur.
Unlike traditional methods that rely on historical data, AI algorithms can instantly adjust forecasts and analyze current market trends, consumer behaviors, and external factors such as weather conditions or economic shifts.
This real-time processing helps companies to be more agile, reducing the risk of overstocking or stockouts.
Adaptive Learning for Demand Forecasting
AI systems employ machine learning techniques that enable them to learn from past data and continuously improve their forecasting accuracy.
These models adapt over time, becoming more refined and accurate as they process new data.
This adaptive learning capability is crucial for industries with volatile demand patterns and unpredictable factors.
AI models constantly adjust their parameters to provide up-to-date forecasts for market conditions.
Integration with IoT for Inventory Management
Integrating AI with IoT devices has opened new avenues for inventory management. IoT devices can track inventory levels, monitor goods conditions, and even predict equipment maintenance.
When fed into AI systems, this data can dramatically improve the accuracy of demand forecasts.
For instance, sensors in a retail store can track foot traffic and product interactions, providing data that AI uses to accurately predict future product demand.
What Factors Affect the Accuracy of AI-based Demand Planning?
Factors that affect the accuracy of AI-based demand planning are data quality, model selection, and integration with the existing IT infrastructure.
Integrating Artificial Intelligence (AI) into demand planning processes is a significant leap from traditional forecasting methods.
AI’s ability to analyze vast amounts of data, including external factors, enables the identification of complex patterns that human analysts might miss.
This capability leads to more adaptive and comprehensive forecasts, helping businesses navigate market complexities, optimize inventory management, and achieve more effective supply chains with reduced risks and lower costs.
However, several critical factors influence AI’s effectiveness in demand planning, including data quality, model selection, and integration with existing IT infrastructure.
Understanding and mitigating the risks associated with these factors is crucial for harnessing the full potential of AI in demand planning.
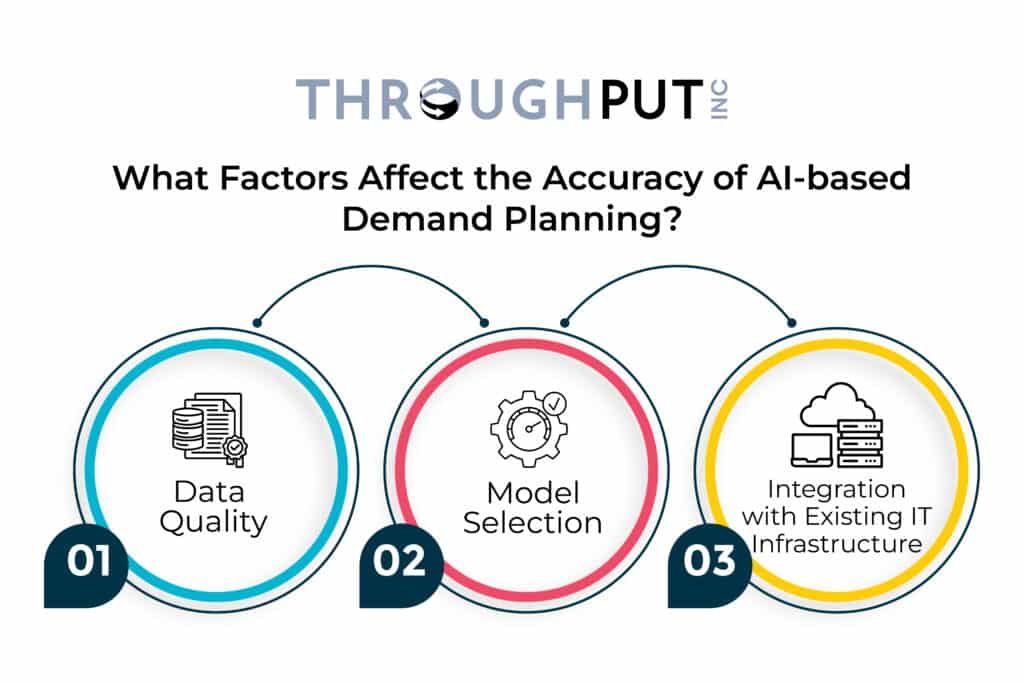
Data Quality
The accuracy of AI-driven demand planning relies on the data quality fed into AI models.
High-quality, integrated data from various sources, including historical sales, market trends and external factors like weather conditions or economic indicators, are essential for generating reliable forecasts.
Poor data quality, inaccuracies, inconsistencies, or incompleteness can lead to misleading AI predictions.
Mitigating this risk involves investing in robust data management practices and consistently cleaning, validating, and updating data.
Model Selection
Choosing the appropriate AI model is another critical factor affecting demand planning accuracy.
The selected model must align with the specific characteristics of the business’s demand patterns and data structure.
While some models may excel in capturing seasonal trends, others better suit products with irregular demand. Employing the right model can result in accurate forecasts, leading to overstocking or stockouts.
Businesses should conduct thorough testing and validation to identify the most suitable model, considering model complexity, interpretability, and computational requirements.
Integration with Existing IT Infrastructure
Integrating AI-based demand planning systems with a company’s IT infrastructure is crucial for seamless data flow and implementing AI-generated insights into operational processes.
Challenges in integration can create silos, hinder real-time data sharing, and reduce the responsiveness of the supply chain.
In order to overcome these challenges, businesses should prioritize the compatibility of AI solutions with their current systems and plan for potential upgrades or adjustments to their IT infrastructure.
Collaboration with IT specialists and AI solution providers facilitates smooth integration.
How to Implement AI Demand Planning in Supply Chains?
Implementing AI demand planning in supply chains involves strategic steps, from initial data collection to full integration and continuous improvement.
This process enhances the accuracy and efficiency of demand forecasts and ensures that the AI system is effectively adopted across the organization.
Here’s a step-by-step guide on how to implement AI in demand planning within supply chains:
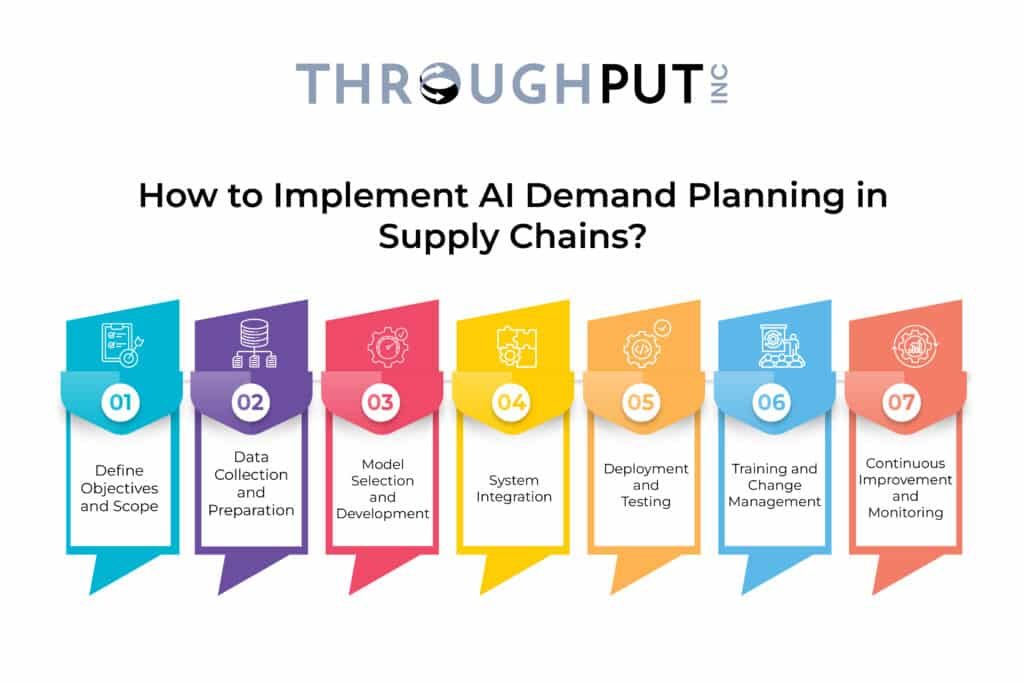
Step 1: Define Objectives and Scope
Clearly defining the aims of artificial intelligence (AI) support is crucial in demand planning for business needs. This includes planning for inventory management, production, or finances.
Decisions like facilitating product demands, lowering out-of-stock, or optimizing stock entry must be made before the AI implementation.
Step 2: Data Collection and Preparation
Compile pertinent information by assembling records of purchases, items in stock, offers, and any external elements that may impact consumption. Refine and prime the information to guarantee correctness and uniformity.
This involves addressing instances of absent data, eliminating atypical elements, and standardizing the data.
Step 3: Model Selection and Development
Determine the suitable AI and machine learning models that match the traits of the demand data. Regression models, neural networks, and time series forecasting models are popular picks.
Establish and Educate the Models by utilizing past data to construct them and ascertain their precision through cross-validation and other desired methods.
Step 4: System Integration
Successfully incorporating an AI system and ensuring it can work well with current IT structures such as ERP, CRM, and supply chain management programs is important.
Automating data processing within and outside the AI system is crucial so data can be gathered and responded to instantly.
Step 5: Deployment and Testing
Before fully implementing the artificial intelligence system, testing it in a contained setting or within a certain part of the logistics process is wise to observe how it performs and make changes as needed.
After acquiring reliable results, the system can be expanded to affect the entirety of the supply chain.
Step 6: Training and Change Management
The workforce must be instructed to operate the recently installed technology, decipher any generated data, and integrate such outputs into their business determinations.
Simultaneously, managers need to handle employee opposition by outlining the advantages of the Artificial Intelligence (AI) infrastructure and ensuring that influential individuals are part of the design and implementation procedure.
Step 7: Continuous Improvement and Monitoring
To keep the AI system in check, it is important to regularly examine a set of predefined KPIs that span over forecast accuracy, inventory levels, and service levels.
With user feedback and data insights, refining and perfecting AI models becomes a never-ending process of improvement, which should continue to enhance its integration with all supply chain operations.
Importance of Change Management
Change management is crucial in the adoption of AI in demand planning. It involves preparing, supporting, and helping individuals, teams, and organizations make organizational change.
Supply planners are advised to uphold open communication where all parties are briefed on the upcoming changes, why they are happening, and how they may benefit.
Additionally, encourage employees to participate in the transitional process to gain their perspective and feel engaged.
Lastly, offer prime support and guidance during the transition and in the aftermath to combat any unanticipated issues effectively and ensure seamless execution.
AI-based Demand Planning with ThroughPut AI
Adopting an integrated approach to planning can prove invaluable for businesses seeking to maximize profit margins.
ThroughPut AI Software Suite, built to embody the principles of Machine Learning alongside those of Deep Learning, offers true end-to-end visibility.
With greater visibility comes the ability to predict trends with increased accuracy, leading to equally precise demand forecasting and planning.
As a result, supply chain disruptions and delays can be more readily identified and addressed to achieve desired bottom-line outcomes.
Book a free demo today to learn more about ThroughPut’s AI Software Suite and how it can improve your business outcomes.
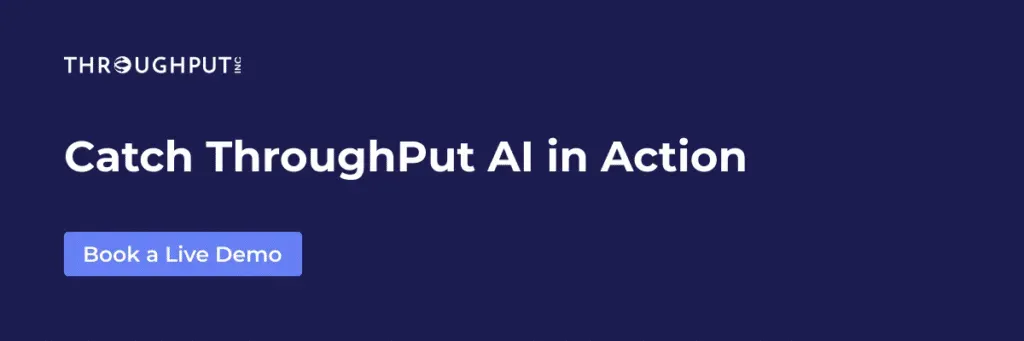