For supply chains, COVID-19 marked the beginning of a challenging era. Even as the immediate pandemic effects stabilized, new challenges like supply shortages, port congestions, and natural calamities emerged. Amidst this ongoing turmoil, one thing that a lot of supply chain professionals have been falling back on (with confidence!) is advanced supply chain analytics.
Advanced supply chain analytics is turning the current uncertainty into strategic foresight. This technology sifts through complex data to forecast potential disruptions and market trends, allowing companies to plan ahead instead of reacting in the moment. It’s not just about responding to immediate challenges; it’s about anticipating them and strategizing accordingly.
In this blog, we’ll delve deeper into the potential of advanced supply chain analytics and understand why it is the need of the hour.
Key Challenges in Supply Chain Management without Advanced Supply Chain Analytics
To understand the power of advanced supply chain analytics, it is important to understand what supply chain management looks like without it.
Reactive vs. Proactive Decision-Making
- Without Advanced Analytics: Decisions are often made in reaction to events as they happen. For instance, if a supplier suddenly runs out of a crucial component, a company might only find out when the delayed shipment fails to arrive, leading to production hold-ups.
- With Advanced Supply Chain Analytics: Advanced analytics can foresee such supply shortages by analyzing patterns, trends, and external factors, allowing the company to source alternative suppliers or adjust production schedules in advance.
Blind vs. Clear Demand Forecasting
- Without Advanced Analytics: It’s hard to predict what customers will want. This can lead to either stockpiles of unsold products or, conversely, empty shelves and missed sales opportunities.
- With Advanced Supply Chain Analytics: Advanced analytics enables demand sensing which generates short-term and mid-term forecasts based on the current realities of the supply chain, reducing errors in forecasting and subsequent upstream challenges.
Static vs. Dynamic Inventory Management
- Without Advanced Supply Chain Analytics: Inventory management might be based on static rules or historical sales data, potentially leading to overstocking or stockouts. A company might order the same quantity of a product each month, not accounting for seasonal demand variations.
- With Advanced Supply Chain Analytics: Inventory levels are dynamically adjusted based on real-time data and predictive models, reducing the risk of excess inventory or stockouts and optimizing storage costs.
Sluggish vs Optimized Transportation and Logistics
- Without Advanced Analytics: Logistics operations may operate on static routing and scheduling, not accounting for variables like traffic, weather, or sudden changes in demand. This can lead to delays, increased costs, and inefficient use of resources.
- With Advanced Supply Chain Analytics: Advanced analytics can optimize routing and scheduling in real-time, considering various dynamic factors. This leads to reduced transportation costs, improved delivery times, and a more agile response to unexpected events or changes in demand.
In each of these areas, the transition from traditional practices to advanced analytics-driven approaches marks a significant shift towards more informed, responsive, and efficient supply chain management. This evolution is fundamental for businesses aiming to thrive in an increasingly complex and unpredictable market landscape.
Benefits of Advanced Supply Chain Analytics for Supply Chain Management
The benefits of advanced supply chain analytics are multi-fold for the current and future supply chain operations. Some of the significant improvements you can expect are:
Agile and Responsive Decision-Making: The real-time data and predictive insights provided by advanced analytics enable supply chain managers to make quick, informed decisions. This agility is crucial for responding effectively to market changes, supply chain disruptions, or unexpected shifts in demand.
Data-Driven Risk Management: Advanced supply chain analytics help in identifying potential risks and vulnerabilities in the supply chain, from geopolitical risks to supplier financial stability. This allows companies to proactively manage risks and develop contingency plans, reducing the impact of disruptions.
Competitive Advantage: Companies that effectively leverage advanced supply chain analytics can gain a significant competitive edge by being more efficient, agile, and customer-focused than their peers who rely on traditional supply chain management practices.
Increased Operational Efficiency: By optimizing routing, inventory levels, and production schedules based on real-time data and predictive insights, companies can significantly reduce operational costs, minimize waste, and improve resource utilization.
Advanced Supply Chain Analytics Techniques
So, what are the advanced supply chain analytics techniques showing immense potential in the face of disruption?
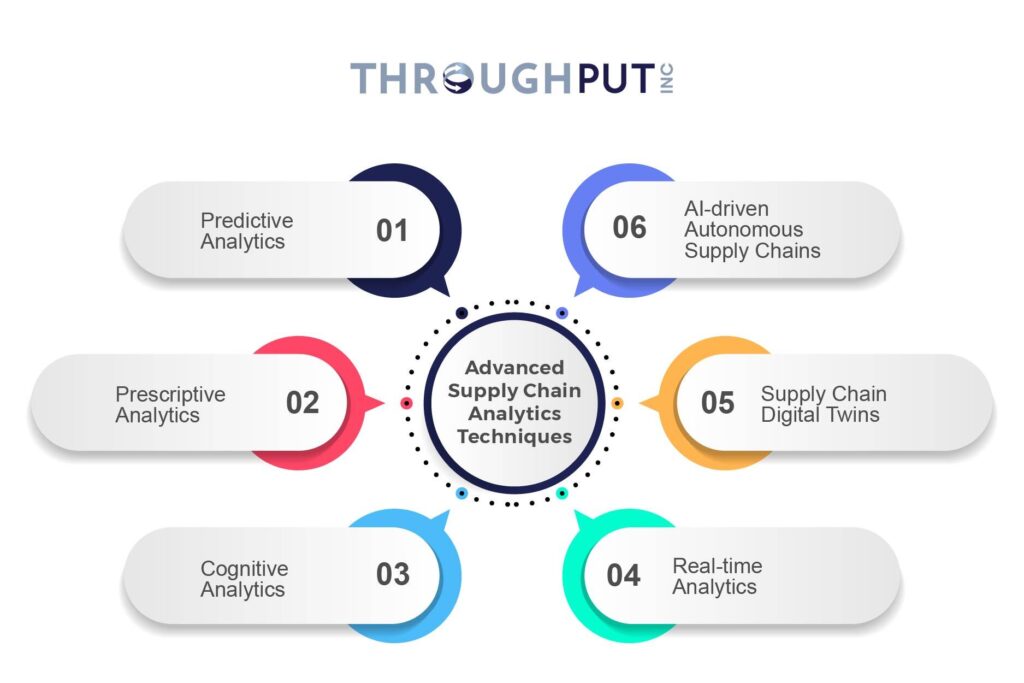
Predictive Analytics (Forecasting and Anticipating)
This technique has become a linchpin in supply chains, employing statistical and machine learning models to forecast demand, inventory needs, and potential disruptions. Predictive analytics shifts organizations from reactive to proactive, basing decisions on foresight about future supply chain events.
Prescriptive Analytics (Optimization and Recommendations)
Going a step beyond, prescriptive analytics not only forecasts the future but also suggests specific actions to streamline supply chain operations. Whether it’s choosing optimal shipping routes, adjusting inventory levels, or setting production schedules, it leverages predictive insights to recommend the best course of action.
Cognitive Analytics (AI and Machine Learning)
Cognitive analytics integrates Artificial Intelligence (AI) and Machine Learning (ML) to process extensive data sets, detect intricate patterns, and adapt by learning from new data. This advanced form of supply chain analytics offers agility and adaptability, setting new benchmarks for data-driven decision-making.
Real-time Analytics (IoT and Sensor Data)
The integration of IoT has propelled real-time analytics to the forefront. Sensors embedded throughout the supply chain gather continuous data, enabling instantaneous adjustments to variables like temperature changes, equipment performance, or shipment tracking.
Supply Chain Digital Twins
The supply chain digital replicas of physical supply chains enable organizations to simulate scenarios, offering a sandbox to predict the outcomes of decisions or disruptions, and plan accordingly before they manifest in reality.
AI-Driven Autonomous Supply Chains
Representing the zenith of supply chain analytics, these autonomous systems employ AI and ML to make real-time decisions and undertake actions independently, adjusting operations dynamically in response to evolving conditions, all with minimal human intervention.
It won’t be an exaggeration to say that a lot of innovation and breakthroughs in supply chain in the future will be a result of the advancements in the field of supply chain analytics. However, for organizations to ride on the possibilities of this technology, data or information management will become an important concern.
Information Management in Advanced Supply Chain Analytics
Information Management is an integral part of advanced supply chain analytics, as it deals with handling vast amounts of data from various sources and transforming it into actionable insights. Here’s a detailed look into various aspects of this domain:
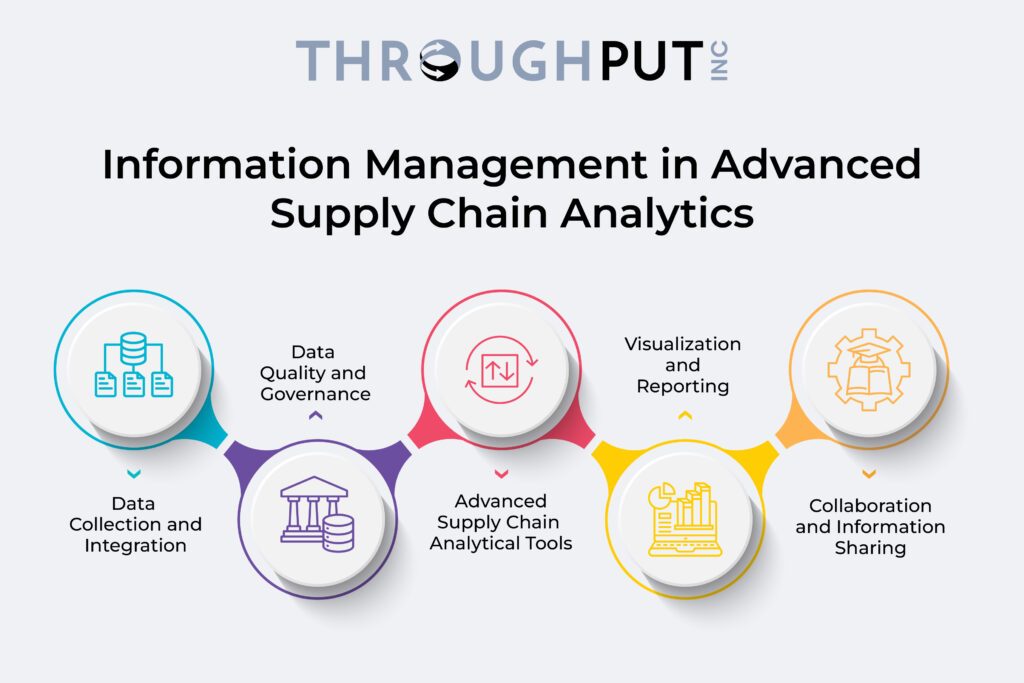
- Data Collection and Integration: The foundation of effective information management and subsequent analytics is the gathering of accurate and comprehensive data from various sources within the supply chain. This includes data from suppliers, production facilities, logistics services, and customer feedback. Integrating this data into a unified system is crucial for a holistic view of the supply chain.
- Data Quality and Governance: Ensuring the quality and consistency of data is paramount. Data governance policies need to be established to maintain data integrity, accuracy, and security. Regular audits and validation processes are essential to ensure that the data used for analytics is reliable and up-to-date.
- Advanced Supply Chain Analytical Tools: Employing sophisticated analytical tools is essential for extracting meaningful insights from complex data sets. Tools powered by techniques that we discussed earlier such as predictive analytics, cognitive analytics and digital supply chain twin can do what may manually be impossible for us to achieve.
- Visualization and Reporting: Complex data sets need to be translated into understandable and actionable formats. Visualization tools and dashboards are crucial for presenting data in an accessible manner, allowing stakeholders to grasp intricate insights at a glance and make informed decisions promptly.
- Collaboration and Information Sharing: Effective information management involves not just internal data handling but also the sharing of information with partners, suppliers, and customers. Secure and efficient collaboration platforms ensure that all parties have access to relevant data, facilitating synchronized supply chain operations and fostering strong partnerships.
Real-World Examples of Advanced Supply Chain Analytics
According to Gartner, by 2024, 50% of supply chain companies will have adopted software with advanced analytics capabilities. Here’s a look at some real-world examples and applications of supply chain analytics.
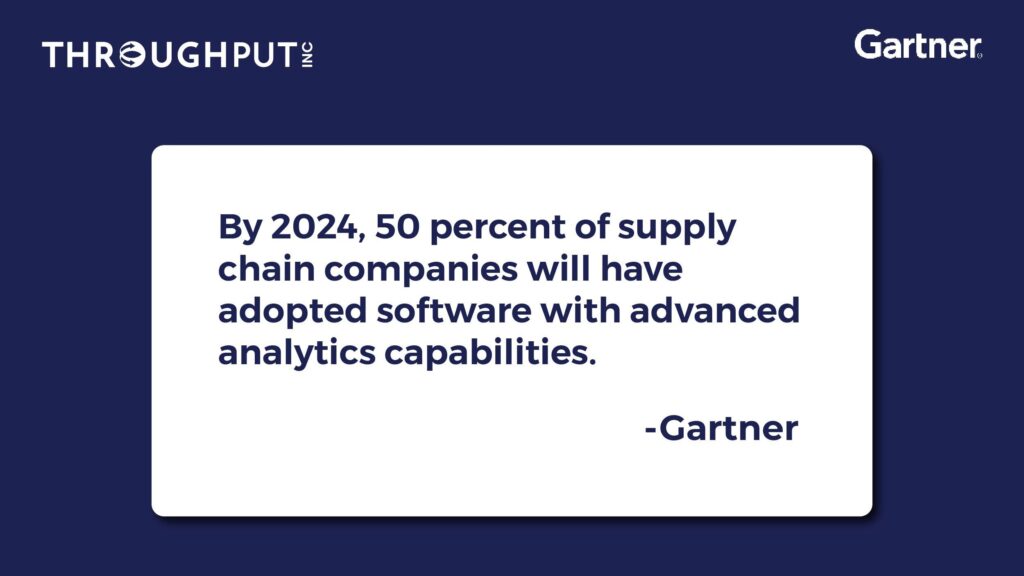
- Cisco
In 2016, Cisco effectively managed the threat of a typhoon to its transportation hubs using advanced supply chain analytics. This allowed them to identify at-risk shipments, notify customers, and protect deliveries during the natural disaster.
- Franprix
Franprix, a French retailer, adopted AI and machine learning-based demand forecasting to optimize stock replenishment. This resulted in a 67% reduction in stockouts and a 30% decrease in fresh food waste, improving inventory management.
- Amazon
Amazon utilizes predictive analytics to optimize its inventory management. By analyzing customer behavior, purchase histories, and search trends, Amazon predicts future product demand. This allows the company to stock inventory intelligently, minimizing storage costs and ensuring products are available for quick delivery, thus enhancing customer satisfaction.
- Starbucks
Starbucks uses an integrated supply chain system that combines mobile data, predictive analytics, and blockchain to streamline its operations. This system tracks coffee beans from farms to stores and predicts inventory needs for each location. By ensuring transparency and efficiency in its supply chain, Starbucks maintains the quality of its products and optimizes its inventory levels.
These examples underscore the transformative potential of advanced supply chain analytics. By leveraging data, AI, and machine learning, companies can gain profound insights into their operations, enhance decision-making, and adapt swiftly to market dynamics, ultimately securing a competitive edge in their respective industries.
Most Common Challenges and Their Solutions in Implementing Advanced Supply Chain Analytics
Implementing advanced supply chain analytics can be a complex endeavor, often fraught with challenges. However, understanding these challenges and adopting strategic solutions can pave the way for successful implementation and sustained benefits.
Here are some of the most common challenges and their corresponding solutions:
- Challenge #1: Data Integration
Many companies grapple with data integration issues stemming from multiple ERP systems across their sites, resulting in data disparities and non-standardized reports.
Solution: Advanced analytics-led platforms streamline data integration by securely connecting to multiple ERP systems. They provide standardized data in a centralized platform, eliminating complex integration efforts.
- Challenge #2: Excel Dependency
Over-reliance on Excel continues due to concerns about data reliability in complex ERP environments.
Solution: Modern supply chain analytics solutions replace Excel dependence with cloud-based collaboration. Real-time insights reduce manual data handling, enabling teams to focus on strategic decisions.
- Challenge 3: Data Quality Issues
Maintaining high data quality poses a continuous challenge, often leading to reactive problem-solving.
Solution: Robust supply chain analytics platforms can automate data quality improvement, identifying and addressing high-value data issues with precision, ensuring accurate and reliable data.
- Challenge 4: Cost Efficiency
Implementing advanced analytics tools can incur substantial upfront costs, including change management and the need for specialized expertise.
Solution: Leading analytics platforms offer cost-effective solutions with pre-built models and analytics. A simple subscription fee makes advanced analytics adoption financially feasible.
Best Practices for Implementation of Advanced Supply Chain Analytics
That brings us to the last question – what are the best practices while implementing advanced supply chain analytics?
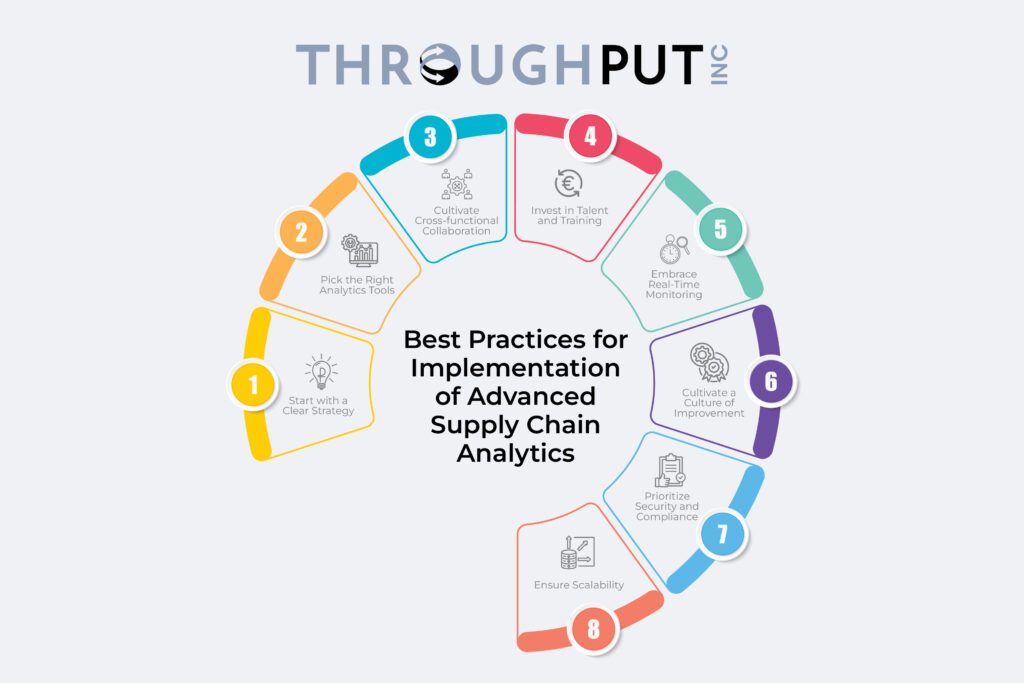
- Start with a Clear Strategy
Begin your journey with a well-thought-out strategy. Define precisely what you aim to achieve, be it optimizing inventory, refining demand forecasts, or bolstering supplier relationships.
- Pick the Right Analytics Tools
The right tools can make all the difference. Choose advanced analytics solutions that align seamlessly with your unique objectives.
- Cultivate Cross-functional Collaboration
Encourage different departments, from supply chain and procurement to finance and IT, to work in harmony. Shared data and insights lead to more comprehensive decision-making.
- Invest in Talent and Training
Your team is your greatest asset. Invest in talent with data science and analytics skills. Elevate your existing staff’s abilities through targeted training.
- Embrace Real-Time Monitoring
Implement real-time monitoring of your supply chain. This allows you to identify and respond to disruptions promptly, reducing the impact on operations.
- Cultivate a Culture of Improvement
Embrace a culture of continuous improvement. Regularly review and refine your analytics models and strategies to adapt to changing market conditions.
- Prioritize Security and Compliance
Protect what matters most—your data. Prioritize robust security measures and compliance with industry regulations, especially when dealing with sensitive information.
- Ensure Scalability
Prepare for the future by ensuring your analytics tools can effortlessly grow with your evolving data needs. Scalability ensures you’re never held back by limitations.
In Conclusion
Advanced supply chain analytics has emerged as a potent weapon in this data-intensive landscape. As the world braces for a future filled with uncertainties, supply chain analytics offer resilience, equipping businesses with the tools to predict, adapt, and thrive.
However, the journey toward advanced supply chain analytics is not without its hurdles. By partnering with experts like Throughput, organizations can harness the power of data to navigate their supply chains confidently through the uncharted waters of the future.
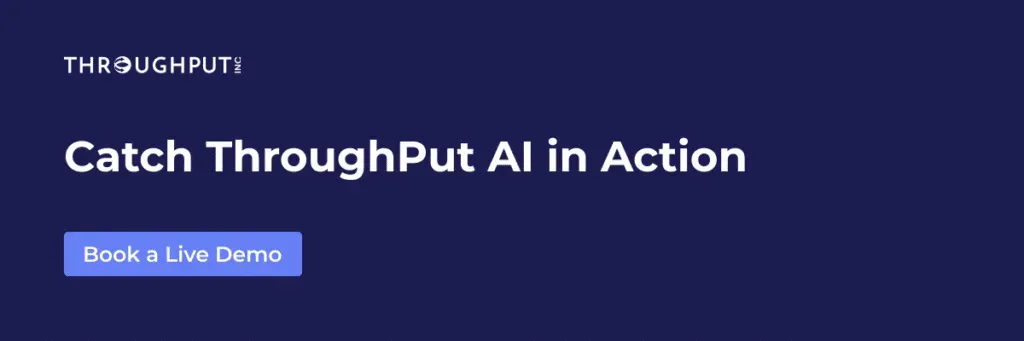