Supply Chain Analytics: The Complete Guide for Improving Supply Chain KPIs
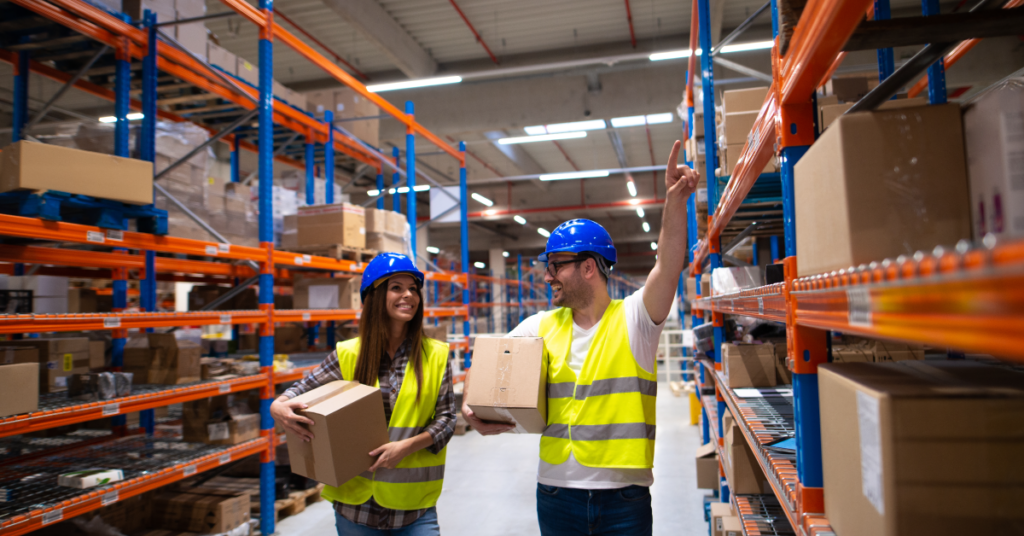
From Google Maps to product recommendations on Amazon, movie recommendations on Netflix, social media feeds and fitness trackers, data and analytics is just about everywhere in life and also, business.
In fact, with the growing volumes of data and technology that enables faster and smarter analytics, this field is changing the way we make decisions everywhere including the supply chain.
With growing disruptions and uncertainty in the supply chain world, the value and benefits of supply chain analytics is only growing further. So for those of you who are just embarking on the analytics journey or are somewhere in the middle but are looking for some insights, this blog is for you.
We prepared this complete guide to help you understand:
- Different aspects of supply chain analytics,
- Its importance,
- How using data mitigates risks and,
- Software solutions and tools that have become must-have technologies.
Let’s dive in.
What is Supply Chain Analytics?
Supply chain analytics, in the simplest terms, is the bridge between data and decision-making. It is the process of analyzing large amounts of data to identify patterns and uncover insights for informed decision-making in supply chain management.
There are currently three core components to supply chain analytics:
- Data analytics
- Data visualization
- Technology platform
Data analytics basically facilitates the extraction of valuable insights from large volumes of data. Data analytics is what is at the core of supply chain analytics but that’s not where it ends.
Data visualization is another key component of smart supply chain analytics. It enables a better understanding of these insights through graphical representations like dashboards, diagrams and more.
And because data is so vast and analysis isn’t always completely possible by humans, these processes are carried out with the help of a technology platform that captures, stores, and analyzes supply chain transactions done by businesses to deliver apt insights and recommendations.
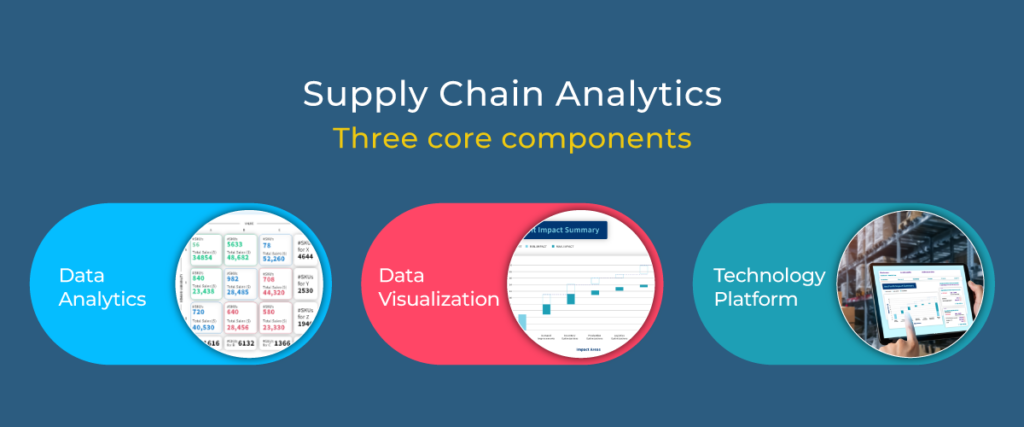
If you were to ask us, we’d say: the primary goal of supply chain analytics is to improve decision-making across strategic, tactical, and operational processes that are a part of a company’s supply chain management. Using data from different applications, infrastructure, third-party sources, and emerging technologies such as the Internet of Things (IoT), supply chain analytics synchronizes supply chain planning and execution, improving real-time process visibility and impacting the company’s bottom line.
Why Is Supply Chain Analytics Important for Companies?
In today’s disruptive and uncertain market landscape, supply chains need to be more agile, fast and responsive. While there are a number of ways organizations are trying to achieve this, supply chain analytics is proving to be the most promising solution of them all.
Here are the key reasons why companies are turning to supply chain analytics:
- We could be repeating ourselves but this is an essential point to reiterate: Supply chain analytics help organizations make better, faster and more informed decisions about their supply chain operations.
- By analyzing data from customers and suppliers, supply chain analytics helps with optimizing inventory management. Analytics can help businesses predict future demand, optimize inventory levels, minimize stockouts, and reduce excess inventory. Let’s not forget that one of the biggest costs for the supply chain is its inventory.
- Supply chain analytics can identify inefficiencies, waste, and areas for improvement – organizations can use the data to streamline their operations, reduce costs, and improve overall efficiency.
- Supply chain analytics analyzes customer data to better predict an organization’s future demand, production planning, procurement, and distribution effectiveness.
All the above establishes how valuable supply chain analytics is to supply chain management. But you need to know one thing without which any amount of analytics can be a failure and that’s data.
What Is the Role of Data in Supply Chain Analytics?
Your analytics is only as good as your data. And by that, we don’t just mean the ‘volume’ of data but its quality and accessibility as well.
Hence, having a good data management practice is integral to effective supply chain analytics and should we also say is your starting point for informed decision-making.
Let’s understand data management a little further.
Importance of Data Management
Data management is vital in supply chain analytics – that’s established. What it does is, it ensures the accuracy, integrity, and availability of data for analysis.
Implementing proper data management practices helps in minimizing errors and inconsistencies and making reliable supply chain decisions based on accurate information.
Overview of Different Types of Data Used
An important aspect of data management is understanding the types of data required for supply chain analytics. Some of the key types of data are:
- Customer data that provides valuable information about consumer preferences, buying behavior, and demand patterns. Analyzing customer data can shape supply chain strategies to better meet customer needs. This information can come from sales records, CRM, invoices and receipts, customer feedback forms and more.
- Product data includes information about the characteristics, specifications, and attributes of different products. Analyzing product data is important for optimizing inventory, demand planning, and forecasting. You can access product data from your inventory management systems, ERPs, POS, and more.
- Demand data is historical and real-time information about the demand for products or services. Analyzing demand data assists in predicting future demand and adjusting supply chain operations accordingly. Sources of demand data can include historical sales data, customer orders, point-of-sale systems, industry reports, weather forecasts, external news, and more.
Data Collection, Cleansing and Integration Techniques for Supply Chain Analytics
Having data is not the most difficult step in the supply chain. In fact, there is hardly any supply chain that doesn’t have enough data. The problem is this data sits in siloed systems and different formats without communicating with each other.
In supply chain analytics, proper data collection, cleansing, and integration techniques are essential to ensure the reliability and accuracy of the analysis. Once collected, the data needs to be cleaned, eliminating any errors, duplicates, or inconsistencies.
Finally, data integration involves combining different datasets from multiple sources to create a comprehensive view of the supply chain.
What Are Common Supply Chain Analytics Techniques and Strategies?
Analytics isn’t just of one type. The process of analytics has evolved so much over the years that you may have come across different terms being used in the context of supply chain analytics. Let us break them down for you.
Some of the most common techniques and strategies include:
- Descriptive analytics: Let’s just say that this is the most primitive of all the supply chain analytics techniques. Descriptive analytics is a technique that provides visibility and a source of information across the supply chain by analyzing historical data to understand past performance and trends.
In simple words, descriptive analytics answers “what happened?”
- Diagnostic analytics is a more advanced version of descriptive analytics. It helps companies identify the root causes of issues and inefficiencies in their supply chain by examining the relationships between different variables and factors.
Diagnostic analytics helps you answer “why it happened?”
- Predictive analytics, unlike the former two, uses historical data, statistical models, and machine learning algorithms to forecast future demand, supply, and other variables. Predictive analytics are used in organizations to plan production, procurement, and distribution more effectively.
Predictive analytics answers a crucial question “What will happen?”
- Prescriptive analytics goes a step further than predictive analytics by recommending specific actions that companies can take to optimize their supply chain operations. Prescriptive analytics combine the insights gained from descriptive, diagnostic, and predictive analytics.
With prescriptive analytics, you get the answer to “what should I do?”
- Cognitive analytics, let’s say, is taking shape even as you read this. Cognitive analytics is also the most advanced version of supply chain analytics that leverages artificial intelligence (AI) and machine learning (ML). It expands the capabilities of prescriptive analytics with its ability to handle massive and diverse types of structured and unstructured data and its inherent ability to learn from new data and improve itself.
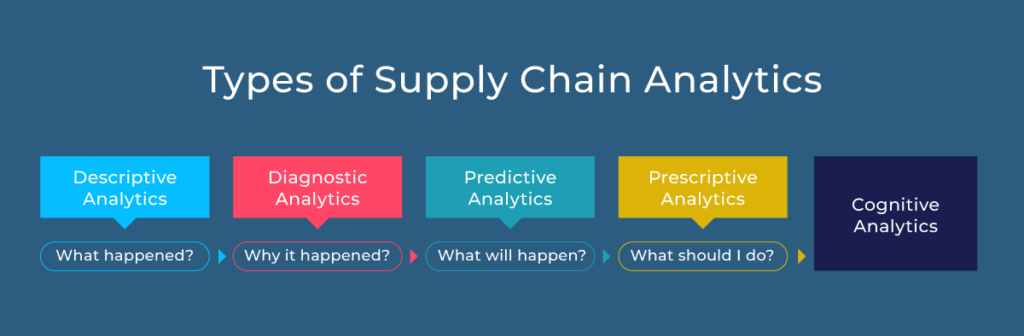
Let’s dig a little deeper into the two powerful forms of analytics: predictive and prescriptive in the next two chapters.
What Role Does Predictive Analytics Play in the Supply Chain?
In two words: A significant role.
Being able to prepare for the future is one of the biggest boons for supply chain professionals in the competitive and unpredictable world.
Here are some specific use cases of predictive analytics in the supply chain:
- Predictive analytics helps businesses with demand forecasting. Companies use predictive systems to optimize their inventory levels and improve operational efficiency by analyzing and forecasting data related to customer demand.
- Route optimization, transportation disruptions, delays, and other logistics-related issues is another example of situations where predictive analytics help immensely. Organizations can optimize their logistics operations to reduce costs and improve overall supply chain efficiency.
- Predictive analytics enables companies to optimize and manage inventory levels, minimize stockouts, and reduce excess inventory by analyzing data from customers and suppliers.
- Maintenance scheduling paired with predictive analytics helps companies schedule maintenance for equipment and vehicles, reducing downtime and improving overall supply chain performance.
- Predictive analytics support supplier risk management by monitoring supplier performance and analyzing data from third-party vendors. The use cases include finding potential risks and disruptions in their supplier network and using proactive measures to mitigate these risks.
- Predictive analytics can support sales and operations planning by providing accurate demand forecasts. The result is better alignment of their supply chain strategies with their sales objectives and improved revenue.
Prescriptive analytics adds another layer over predictive analytics and that is of recommendations.
How Does Prescriptive Analytics Help Supply Chain Professionals?
Prescriptive analytics is predictive analytics + recommendations. And that’s why it plays a vital role in supply chain management because it recommends specific actions that companies can take to optimize their supply chain operations.
Here are some examples to show you how prescriptive analytics helps supply chain professionals:
- A hospital’s supply chain data analytics system predicts a potential flu outbreak in the upcoming weeks based on various external data sources such as regional flu cases, weather patterns, and historical data. This is predictive analytics in action. Perspective analytics will go on to further recommend that the system increase the stock of flu vaccines, adjust staff schedules for anticipated higher patient inflow, and initiate a public health awareness campaign to mitigate the spread and impact of the flu.
- A logistics company predicts that road construction and a local event will cause traffic delays on a primary route. The prescriptive analytics system will then recommend rerouting certain deliveries through an alternate path, adjusting delivery time windows, and notifying customers in advance about potential delays.
- An electric utility company predicts higher energy demand for the upcoming week due to a heatwave. The system suggests optimal pricing strategies to manage demand, advises the activation of additional power generation resources, and recommends communication strategies to encourage consumers to reduce energy usage during peak hours.
In each of these examples, the power of supply chain prescriptive analytics is seen in how it not only anticipates future scenarios but also suggests specific actionable measures to handle or optimize those scenarios.
That’s the potential of prescriptive analytics. So what happens when you add AI to the supply chain analytics mix?
How Can AI Improve Supply Chain Analytics?
Supply chain analytics is promising by itself. Now add AI to that mix and you have the recipe for some powerful decision-making in the supply chain.
Let’s see more clearly what AI brings to the supply chain analytics table:
Handling of Vast and Complex Data: AI can process and analyze enormous datasets, including unstructured data such as text, images, and videos. Traditional analytics often struggled with these data types.
Cognitive Decision Support: AI platforms can offer decision-makers cognitive insights, which are not just data-driven but also consider context, potential implications, and business strategies.
Real-time Analysis and Responses: AI systems can analyze data in real-time, allowing for instant decision-making. This speed is vital in situations where timely responses can prevent stockouts, manage sudden demand spikes, or reroute shipments due to unforeseen disruptions.
Advanced Demand Forecasting: AI can factor in a multitude of variables (like social media sentiment, local events, and even weather patterns) to predict product demand more accurately.
Improved Visibility and Traceability: AI can integrate data across the entire supply chain, from raw material suppliers to end customers, offering a level of transparency and traceability that was hard to achieve previously.
ThroughPut’s AI-powered decision intelligence and analytics combines all the above to enable supply chain decision-makers to make smart, fast and confident decisions that drive business results.
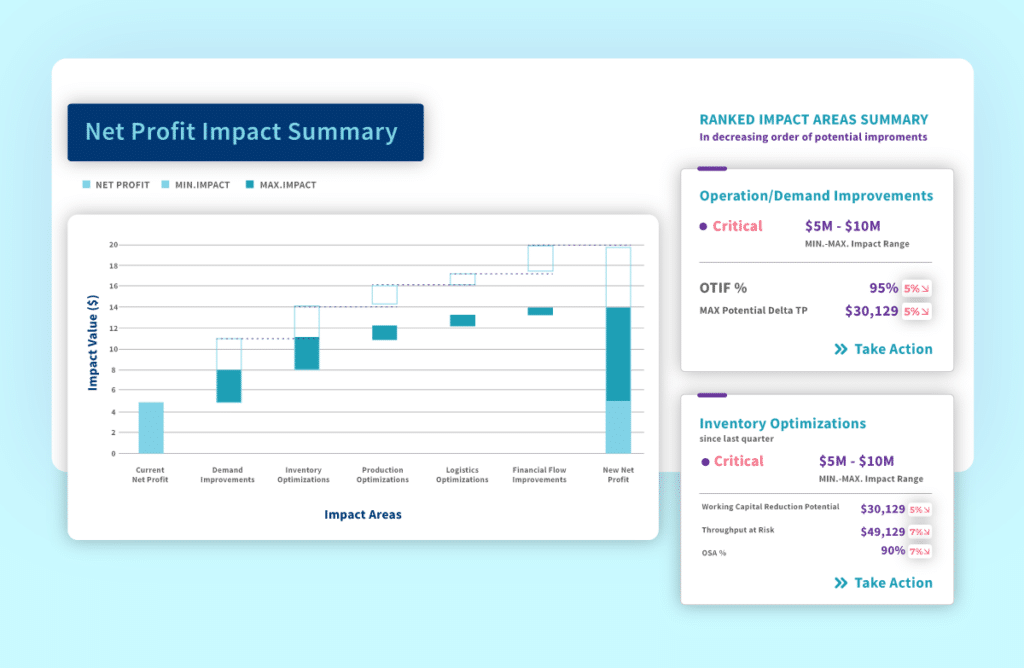
ThroughPut caters to the biggest pain points in the supply chain currently:
- Time-to-value: Supply chains need to show ROI now and ThroughPut does that 5X faster than any other solution out there.
- Outcome-driven approach: When it comes to something as complex as analytics, it is possible to get lost in implementation. But ThroughPut takes an outcome-first approach, solving your biggest supply chain challenges with analytics.
- Contextual & real-time: ThroughPut enables you to make decisions in real-time without losing track of the context.
- Demand Sensing: ThroughPut’s Demand Sensing tool drives the much-needed accuracy in forecasting by considering short and near-term demand.
Supply Chain Analytics Visualizations
We wanted to touch upon supply chain analytics visualizations in this blog because it is visualizations that make the insights accessible to one and all.
One of the most common forms of supply chain analytics visualization is a dashboard. Dashboards contain live data about what is happening within your supply chain. For instance, this screen below shows the stock levels of SKUs, clearly labeling out the ones that are low in inventory and needs to be restocked ASAP.
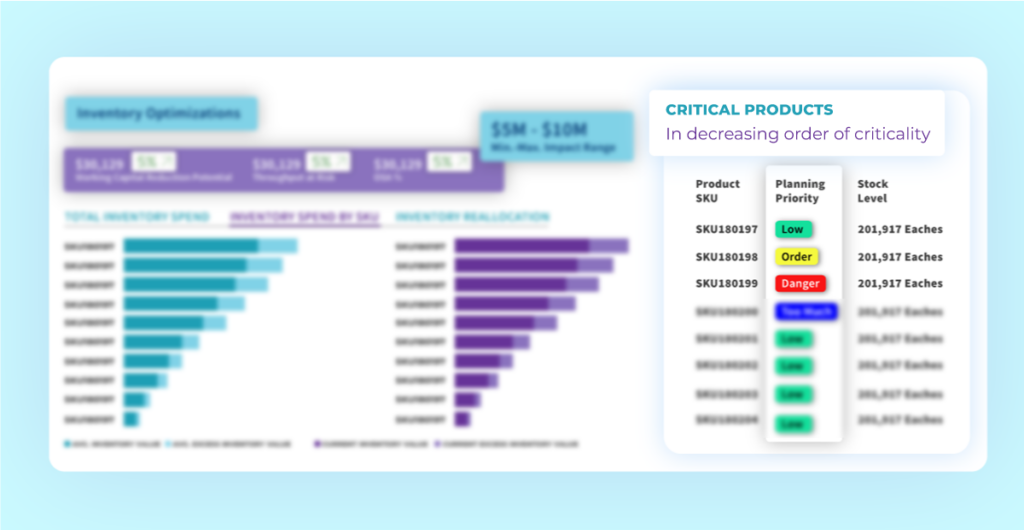
Simply put, supply chain dashboards are reports that show the current status of key performance indicators. Ideally, a dashboard needs to be updated automatically based on live data without manual intervention.
Often, you would want to dig deeper into some of this information. A good dashboard will enable you to delve further into the data. For instance, in the above dashboard, you can click on any of the SKUs to see the historical trends in inventory management of this particular SKU and a predicted trend of what stock need to be maintained based on forecasted demand.
Some of the other forms of data visualizations in supply chain analytics include:
- Time Series Charts: Displays trends over a period, such as monthly sales, yearly inventory levels, or daily shipment deliveries.
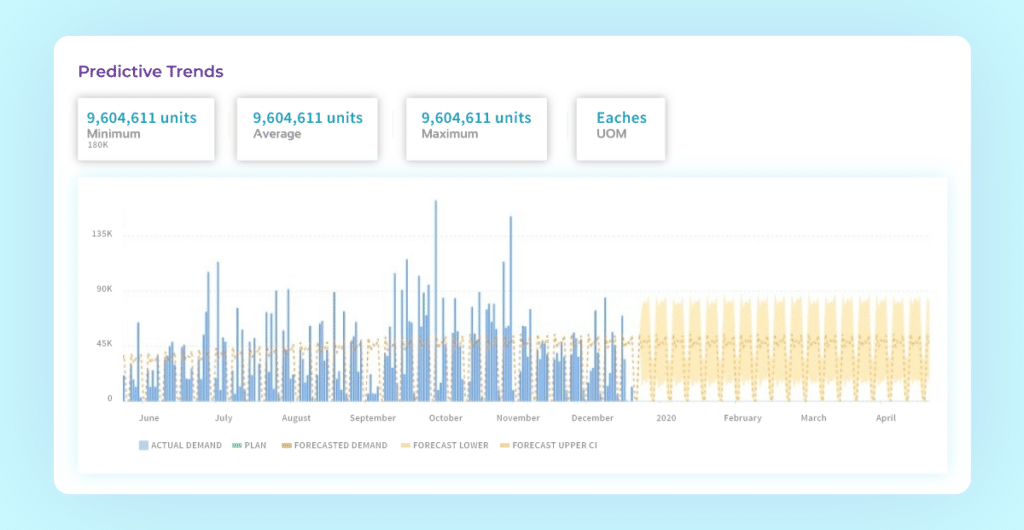
- Heat Maps: Useful for identifying high and low concentrations in a dataset, such as regional sales performance or areas with the highest delivery delays.
- Geographical Maps: Plot supply chain entities like warehouses, suppliers, and customers on a map. These visualizations can highlight transportation routes, shipment status, and regional performance.
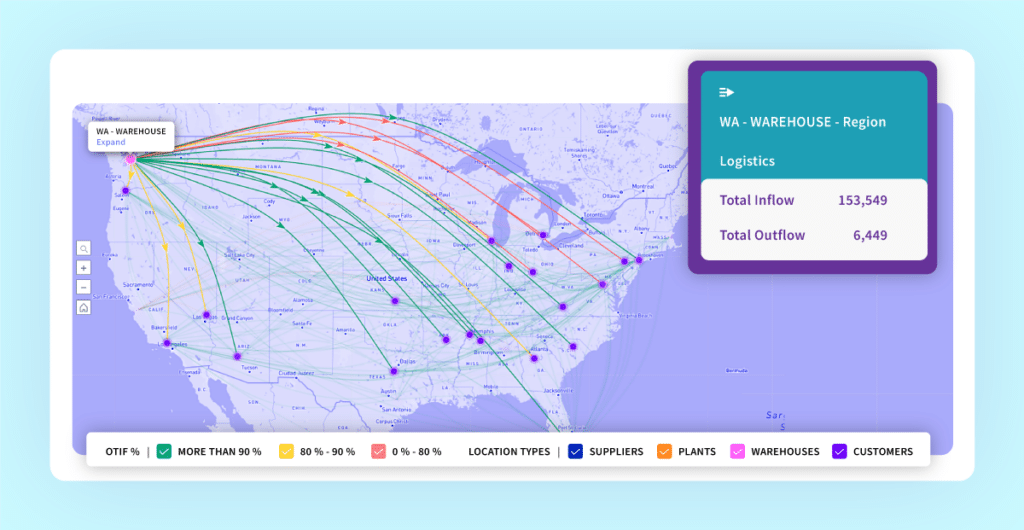
- Scatter Plots: Illustrate the relationship between two variables. For instance, they can show the correlation between delivery times and customer satisfaction.
- Pie and Donut Charts: Break down categorical data, such as the market share of different products or the proportion of different transportation modes used.
- Bar and Column Charts: Compare different categories side by side, like sales performance across various products or regions.
- Gantt Charts: Essential for project management within the supply chain, these charts display the timeline of various tasks, helping in coordinating activities like product launches or warehouse setups.
- Sankey Diagrams: Visualize the flow of goods or information from one part of the supply chain to another, showing the distribution pathways and their volume.
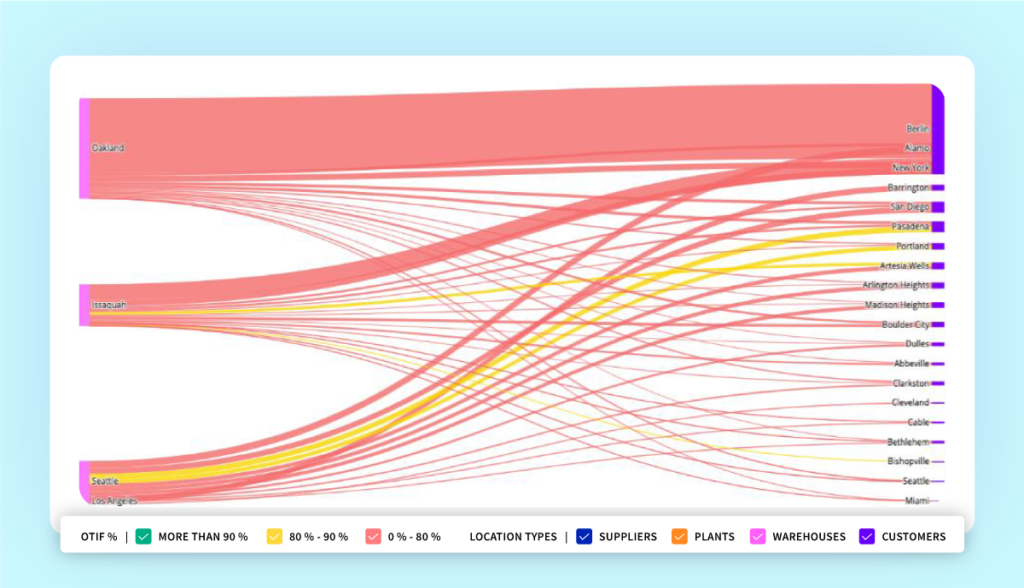
- Tree Maps: Represent hierarchical data and the part-to-whole relationships, like breaking down total sales into product categories and then individual products.
- Bubble Charts: Like scatter plots but with an additional dimension represented by the size of the bubbles. They could show the relationship between product demand, profit margin, and stock levels.
- Box Plots (Whisker Plots): Show the distribution of data into quartiles, highlighting the median, outliers, and potential anomalies. Useful in understanding metrics like delivery time variability.
- Radar (Spider) Charts: Compare multiple metrics across different categories. For instance, evaluating a supplier’s performance based on criteria like delivery time, quality, cost, and responsiveness.
- Histograms: Display the distribution of a continuous dataset, such as the frequency of delivery times or order sizes.
- Control Charts: Monitor the stability or variability of processes over time. They help in quality control by signaling when a process is out of the predetermined control limits.
These visualization tools can allow anyone within your supply chain to quickly comprehend complex data patterns, monitor performance, identify bottlenecks, and make informed decisions.
What Are Some Risks That Companies Can Mitigate by Using Supply Chain Analytics?
Risks abound in the supply chain. Below are the top risks that companies are managing to mitigate with supply chain analytics.
- Demand and supply imbalances: Supply chain analytics help organizations better predict future demand and plan production, procurement, and distribution more effectively – all this reduces the risk of imbalances.
- Supplier disruptions: By monitoring supplier performance and analyzing data from third-party vendors, analytics can help companies identify potential risks and disruptions in their supplier network, allowing them to take proactive measures to mitigate these risks.
- Supply chain analytics can help companies optimize their logistics operations, reducing the risk of delays, transportation disruptions, and other logistics-related issues.
- By optimizing inventory management, supply chain analytics help businesses minimize stockouts and reduce excess inventory, mitigating the risks associated with inventory management.
- Another feature of supply chain analytics is the assessment of the impact of global political unrest, economic fluctuations, and other external factors on a company’s supply chain operations. Thanks to the information, organizations can develop risk-based supply chain plans and strategies.
- As supply chains become more interconnected and reliant on technology, the risk of cyber attacks and data breaches increases. Supply chain analytics assist with identifying potential vulnerabilities and implementing security measures to protect their supply chain operations.
Another prominent use case of supply chain analytics – one that most supply chain leaders have immense interest in today’s times – is cost reduction.
What Are Some Examples of Supply Chain Analytics Reducing Costs?
Several companies have successfully implemented supply chain analytics to reduce costs and improve their operations. Here are some notable examples of successful implementation from different industries:
- Walmart has implemented a range of cost-reduction initiatives, including inventory management and transportation optimization, which have helped the company reduce supply chain costs significantly.
- A global cement manufacturer saved $1.5M in working capital costs using advanced supply chain analytics by proactively identifying hidden and unplanned bottlenecks.
- Unicsoft, a software development company, used supply chain analytics to reduce operations costs by improving traceability in the supply chain process, enabling accurate forecasting to meet customer demand, enhancing customer loyalty, and reducing inventory costs.
- An aircraft sensor manufacturer invested in supply chain analytics to maximize its capacity utilization and achieved annual savings of $10 million.
- By optimizing their SKUs and logistics through supply chain analytics, a Retail Company in Europe saved €3.5 Million per Year in Logistics Costs.
These examples demonstrate the potential of supply chain analytics to reduce costs and improve operations. And we have a lot more other examples from ThroughPut’s clients that successfully used prescriptive analytics to optimize their supply chain.
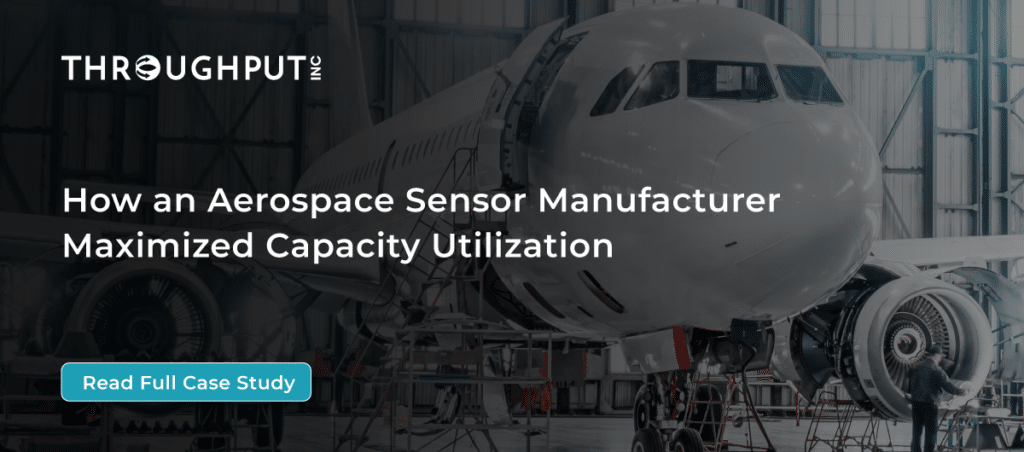
What Are the Best Practices for Implementing Supply Chain Analytics?
Implementing supply chain analytics may not be a cakewalk. But when approached in a planned and scalable way, it isn’t impossible either. We’ve seen numerous examples of data transformation and analytics and here are some best practices for implementing supply chain analytics from our experience.
- Find a sponsor in the C-suite. One person who sees the value in supply chain analytics and is able to get everyone on board to ensure a cohesive approach to analytics implementation.
- Adopt the right technology platform.It is crucial for successful supply chain analytics implementation. Choose a platform that meets internal needs and integrates seamlessly with your existing supply chain processes.
- Concentrate your analytics efforts on areas that have the most significant impact on your supply chain performance, such as demand forecasting, inventory management, and supplier risk management.
- Leverage real-time data to help your organization deal with disruptions and make more informed decisions. Ensure your analytics platform can handle real-time data and provide actionable insights.
- Invest in employee training and development. Your internal personnel need to have the necessary skills and knowledge to work with supply chain analytics tools and successful implementation. Invest in training programs and resources to help your team effectively use analytics in their daily tasks.
- Continuously monitor and evaluate the performance of your supply chain analytics implementation. Identify areas for improvement and make adjustments as needed to ensure optimal results.
- Integrate internal and external data by bringing together data from different applications, infrastructure, third-party sources, and emerging technologies such as the Internet of Things (IoT) to gain a comprehensive view of your supply chain operations.
- Implement lean and agile methodologies to improve supply chain efficiency, reduce waste, and respond quickly to changing market conditions.
- Ensure high-quality data for accurate and reliable analytics insights. Implement data quality management processes to ensure the data used in your analytics is accurate, complete, and up-to-date.
These best practices will help you successfully implement supply chain analytics and leverage its benefits to improve operations and reduce costs.
Challenges and considerations when integrating analytics into existing supply chain management systems
Integrating analytics into existing supply chain management systems can be a complex process with several challenges and considerations. One of the main challenges is ensuring compatibility between the analytics tools and technologies and the existing systems. This requires a thorough understanding of the current system’s capabilities and limitations.
Another consideration is the availability and quality of data. Analytics heavily rely on accurate and timely data to generate insights and make informed decisions. Therefore, your or another organization must ensure that the supply chain data is clean, organized, and easily accessible for analysis.
Furthermore, there is often resistance to change when integrating analytics into existing systems. This can be due to concerns about disruption of existing processes, learning and adopting new technologies, and fear of job displacement. It is important to address these concerns and actively involve key stakeholders throughout the integration process to ensure smooth adoption and implementation.
Ensuring scalability and sustainability of supply chain analytics initiatives
Scalability and sustainability are important considerations when integrating analytics into supply chain management systems. To ensure scalability, organizations should choose analytics tools that can handle increasing volumes of data and complexity as the business grows.
To achieve sustainability, businesses should establish a governance framework that includes data management processes, maintenance plans, and continuous improvement practices. This kind of framework will help ensure that the supply chain analytics initiatives stay relevant and effective over time.
What Are the Best Supply Chain Analytics Tools?
There is no shortage of great supply chain analytics tools. Technology advancements have made it possible to have the best software in class and receive precise and actionable insights at any time.
And while it is hard to define the best solution (because that always depends on the exact needs of a business), some tools have better capabilities than others.
With that said, here is a list of a couple of tools that you should consider when thinking about implementing supply chain analytics in your organization.
- ThroughPut AI
- Palantir Foundry
- SAP Supply Chain Analytics Software
- Oracle Fusion SCM Analytics
- Blue Yonder
- Manhattan Associates
- Tableau
- GMDH Streamline
- IBM Supply Chain Analytics
- Supply Chain Guru X by Coupa
- Kinaxis RapidResponse
- O9 Solutions
- Anaplan
- Qlik
- SAS Supply Chain Analytics
- Aera Technology
- Conversight
- Tredence
As the owners of ThroughPut AI, we may be gently biased, but we strongly believe that this tool offers a comprehensive view of all critical supply chain elements and can transform almost any supply chain dramatically with a keen eye on business value.
Make sure to read our review of the best supply chain analytics software solutions.
What is the Future of Supply Chain Analytics?
Before we end this article, let’s briefly look at how supply chain analytics will evolve in the future.
Without a doubt, the future of supply chain analytics will be shaped by technological advancements, changing business environments, and an ever-increasing need for agility, resilience, and efficiency in supply chains.
Here are some emerging trends and technologies you can expect.
Emerging trends and technologies in supply chain analytics
One main trend will be the integration of advanced technologies like AI, IoT and blockchain to deliver more powerful results through supply chain analytics.
Another trend you can expect to see is that of an autonomous supply chain and automated decision-making. Many supply chains will start to largely operate and optimize themselves based on predefined goals and real-time data.
Analytics for sustainability will gain momentum in the years to come. Supply chain analytics will be used to assess and optimize the environmental footprint of supply chains. This includes carbon footprint tracking, optimizing routes for fuel efficiency, and assessing supplier sustainability practices.
Last but not least, with the rise in the importance of analytics, there will be an increasing demand for professionals skilled in data science, AI, and supply chain management. New roles centered around data-driven decision-making will emerge.
In the next 5-10 years, experts predict that advanced analytics will become even more embedded in supply chain management practices, enabling businesses to achieve unprecedented levels of efficiency and responsiveness.
So how can you make the best of supply chain analytics and stay ahead of the competition?
Strategies for staying ahead of the competition through continuous improvement in supply chain analytics
To stay ahead of the competition, you must continually improve your supply chain analytics capabilities. This involves investing in cutting-edge technologies, such as AI, ML and IoT that can generate actionable insights and enable data-driven decision-making.
Additionally, fostering a culture of continuous improvement and learning is crucial. Supply chain professionals should actively seek opportunities to enhance their analytical skills and stay updated on the latest trends and best practices in supply chain analytics. By regularly assessing and optimizing supply chain processes, you can identify areas for improvement, streamline operations, and gain a competitive edge in the rapidly evolving landscape of supply chain management.
All the best!