Beyond the Hype: 12 Real Challenges of AI in Supply Chain
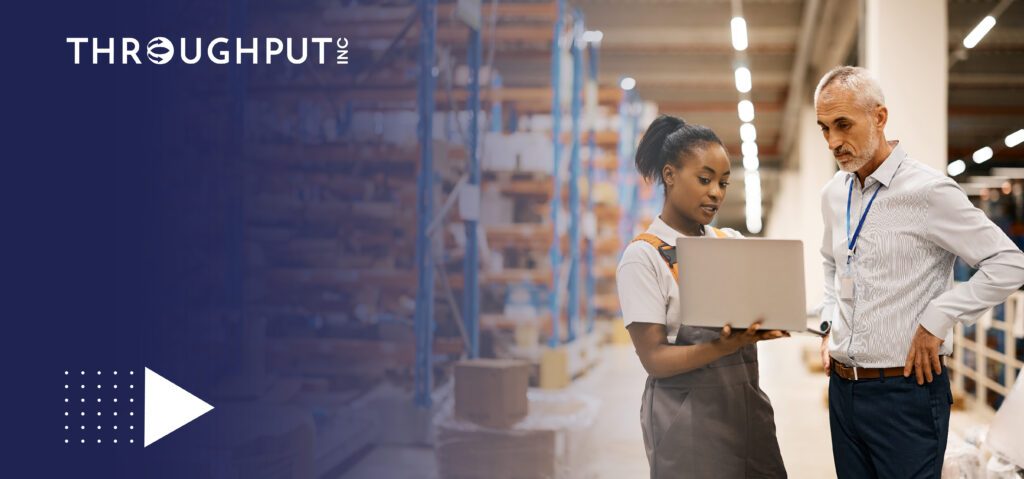
The whole wide world is excited about Artificial Intelligence (AI). In the realm of supply chain management, AI is being heralded as a transformative force, capable of streamlining operations, slashing costs, and enhancing overall efficiency.
There really is no doubt about the potential of AI. If there’s any concern at all, it is surrounding the implementation and adoption of this technology in supply chain operations.
You need to approach AI with a discerning eye.
The enthusiasm for AI in supply chain can sometimes overshadow the reality that it’s not a universal solution. Implementing AI without strategic planning and execution can set you back instead of pushing you forward.
In this blog, we’re going to look closely at 12 challenges of AI in supply chain.
12 Challenges of AI in Supply Chain
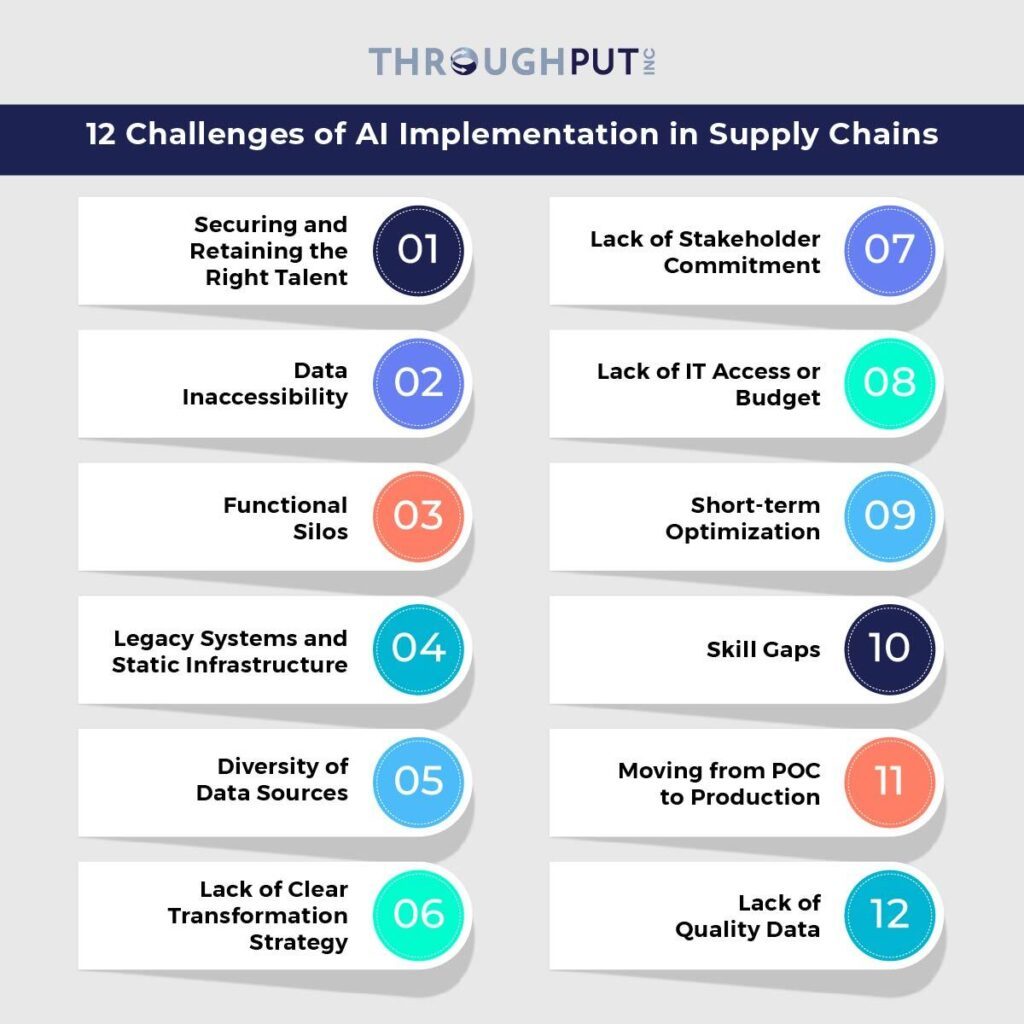
1. Securing and Retaining the Right Talent
Topping the list of challenges is the intense competition for AI talent.
The talent challenges of AI in supply chain isn’t just about recruitment; it’s about nurturing a workforce that can blend AI expertise with supply chain acumen.
In today’s market, AI professionals are in high demand across numerous industries, making the competition (extra) fierce. You must not only attract these rare talents but also invest in their continuous development and integration within the supply chain context.
Ultimately, the success of AI implementation depends heavily on how well these professionals can tailor AI solutions to fit the unique dynamics of your supply chain operations, making their role both challenging and indispensable.
2. Data Inaccessibility
Data inaccessibility is a major challenge for AI in supply chains.
Data acts as the essential fuel for AI where it drives decision-making and operational improvements. If AI cannot access the necessary data, its learning and decision-making capabilities get severely limited. This leads to less accurate predictions and inefficiencies in the supply chain.
Therefore, ensuring easy access to relevant data is crucial for effective AI integration and performance in supply chain management.
3. Functional Silos
The classic silo problem: Teams working in isolation, and not communicating with each other.
Functional silos lead to isolated data and knowledge within separate departments. This isolation prevents AI systems from accessing the comprehensive information they need.
AI thrives on wide-ranging, integrated data for effective analysis and optimization. In a siloed environment, AI can’t get a complete view of the supply chain, reducing its effectiveness.
4. Legacy Systems and Static Infrastructure
The integration of AI in supply chain management is significantly impacted by the presence of outdated technologies. These legacy systems, often entrenched in operational areas, can be expensive and time-consuming to update, leading to inefficiencies and reduced productivity. These systems, while once cutting-edge, now struggle to adapt to the dynamic demands of the modern market.
Modifying or upgrading these legacy systems is neither easy nor economical. It requires significant investment in terms of time, effort, and financial resources. Key issues that need to be addressed include:
- Integrating AI with Existing Systems: Figuring out how to seamlessly incorporate AI into legacy systems without disrupting existing operations.
- Managing High Maintenance Costs: Planning and allocating funds effectively to maintain smooth operations while integrating new technologies.
- Enhancing Flexibility: Overcoming the inherent rigidity of legacy systems to allow for more adaptable and responsive operations.
- Data Management: Tackling the challenges related to organizing, processing, and utilizing data in a way that is compatible with both old and new systems.
5. Diversity of Data Sources
As discussed above, the implementation of Artificial Intelligence (AI) in supply chain management is heavily reliant on data diversity. This reliance on diverse data sources introduces a complex challenge: the need to efficiently manage and process data from various origins, each differing in format, structure, and method of processing.
To tackle this complexity, businesses must adopt a comprehensive strategy centered around diverse data collection and curation. Ensuring that the data feeding into AI models is not just abundant but also varied and representative is crucial.
6. Lack of Clear Transformation Strategy
In the realm of supply chain management, particularly with the implementation of Artificial Intelligence (AI), the necessity of a well-defined strategy is paramount. The absence of a clear strategy can lead organizations astray, leading to misaligned activities with the overall company objectives, resulting in resource wastage and a lack of directional focus.
For effective AI implementation in supply chain processes, it’s essential to develop a vision and strategy that aligns with the organization’s broader goals.
This strategic approach should revolve around customer-centricity, reevaluation of existing supply chain processes, and encouragement of a culture where employees are prepared to adapt to technological changes and upskill accordingly.
7. Lack of Stakeholder Commitment
Stakeholder commitment in AI implementation is far more than a procedural formality; it’s the lifeblood of success. When stakeholders’ views on business operations are misaligned, it can lead to distrust, fear, and a lack of engagement, posing significant challenges to the AI implementation process.
The key to enlivening stakeholder enthusiasm for AI lies in a well-crafted AI transformation strategy. This strategy must focus on securing and maintaining stakeholder buy-in, ensuring that all parties are not just informed but are genuinely interested and invested in the AI journey.
8. Lack of IT Access or Budget
The lack of IT budget or access significantly hampers AI implementation in supply chain, with high initial costs for infrastructure, software, and talent posing a major barrier. Ongoing operational expenses and the rapid pace of technological advancements further strain limited resources, making it challenging for organizations to keep up.
However, adopting cloud-based solutions, utilizing open-source frameworks, and focusing on specific, high-impact areas can help mitigate these financial challenges to a great extent.
9. Short-term Optimization
Prioritizing short-term gains over long-term benefits can significantly impede the progress of AI integration.
A vision that extends into the future is crucial for the successful implementation of AI, ensuring that the technology not only meets immediate needs but also aligns with long-term organizational goals. This foresight fosters an environment where AI can evolve and adapt, ultimately driving innovation and sustained growth.
10. Skill Gaps
The supply chain team needs to possess the right skills to ensure the smooth integration and functioning of AI systems. However, the rapid pace of AI advancement leads to a shortage of professionals who are well-versed in specialized areas such as deep learning and natural language processing.
Identifying and bridging these gaps is essential and involves investing in comprehensive training and development programs. The urgency of this issue is highlighted by a report from Skillsoft, which indicates that 66% of executives rank their team’s proficiency in AI and machine learning as medium to low.
This underscores the pressing need to enhance skills and knowledge in these areas, ensuring teams are equipped to meet the demands of AI implementation and drive successful adoption.
11. Moving from POC to Production
Transitioning from a proof of concept (POC) to full-scale production is indeed a significant leap for any organization. This phase is marked by a substantial increase in data management complexity where concerns like data quality and accessibility take center stage.
As the scale enlarges, issues that seemed manageable during the POC phase, such as inadequate tools, lack of expertise, and suboptimal data management practices, can become pronounced obstacles.
12. Lack of Quality Data
Data quality is the linchpin of successful AI implementation, directly impacting the performance and accuracy of AI models. High-quality data fuels precise predictions and robust insights, while poor data quality can lead to misleading outcomes, undermining the reliability and effectiveness of AI solutions.
Incomplete or inaccurate datasets can cause AI algorithms to overlook critical patterns, leading to skewed or incomplete analyses.
Embracing the Future: Navigating The Challenges of AI in Supply Chain Success
In navigating the complexities of evolving supply chains, the challenges we’ve discussed aren’t mere obstacles but rather catalysts for innovation and progress. Each of the twelve challenges to AI implementation in the supply chain underscores the necessity for a well-orchestrated strategy, turning potential hurdles into stepping stones for enhancement and growth.
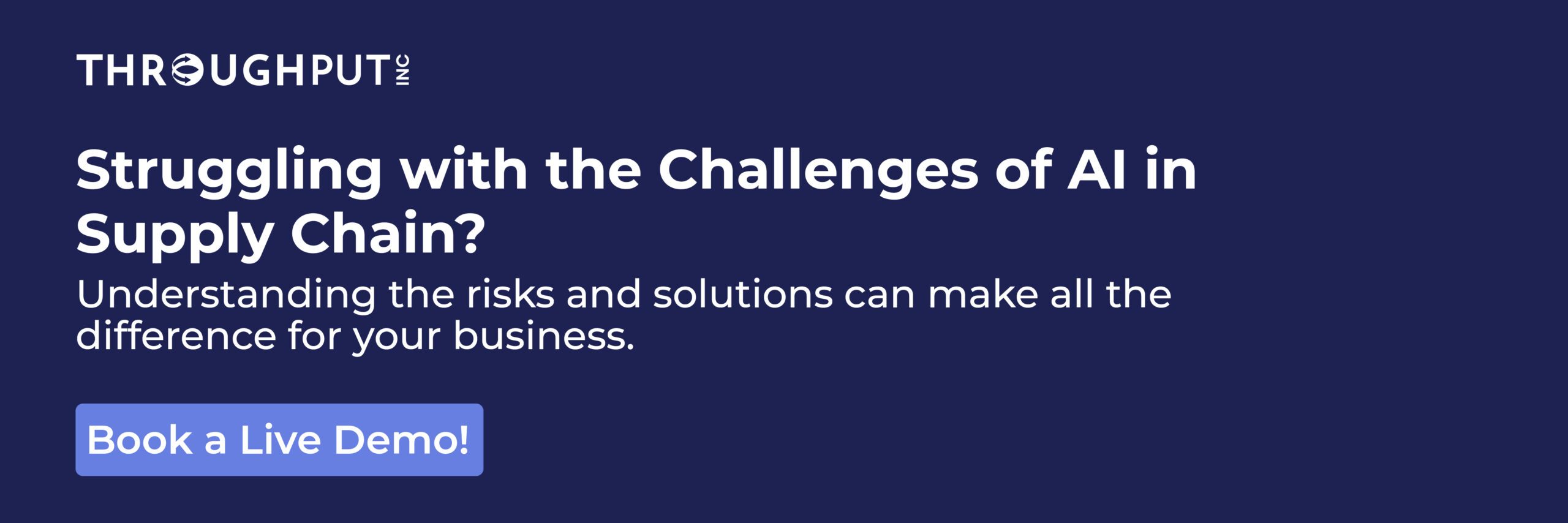