Demand Sensing All-inclusive Guide for 2024
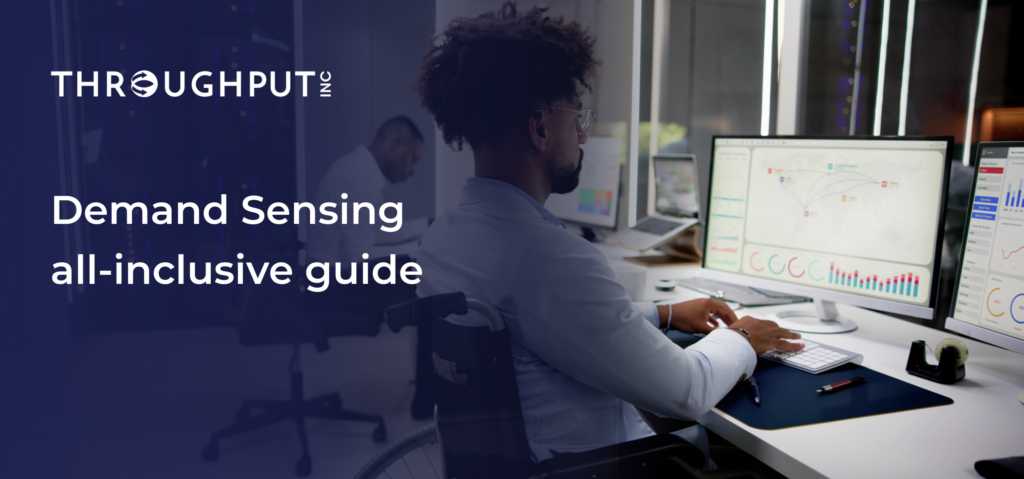
Demand sensing leverages the latest supply chain technology to interpret real-time data and provide the insights needed to make informed decisions swiftly.
This article dives into demand sensing, explaining its meaning, emphasizing its significance, and unraveling its process.
The all-inclusive guide reveals the advantages of utilizing demand sensing in streamlining operations for supply chain experts and planners.
We’ll also highlight demand sensing challenges in its execution.
What is Demand Sensing?
Demand sensing is the process of using real-time data and advanced analytics to understand and predict customer demand.
This method enables companies to optimize their supply chain and inventory management by being more agile and responsive to current market conditions.
Demand sensing differs from traditional forecasting by focusing on the short-term horizon and leveraging up-to-date information on changes in consumer behavior, weather patterns, and economic conditions.
What are the Components of Demand Sensing?
The key components of demand sensing systems are data inputs, analytical tools, and output capabilities.
Data inputs are demand sensing systems that collect a wide variety of data types, including but not limited to point-of-sale (POS) data, online sales data, social media trends, weather forecasts, and economic indicators.
This data is more granular and immediate than the historical sales data used in traditional forecasting, providing a more accurate and current picture of demand.
Analytical tools are advanced analytics, artificial intelligence (AI), and machine learning (ML) algorithms that analyze the collected data to identify patterns, trends, and correlations that might not be apparent through manual analysis or traditional statistical methods to predict demand shifts more accurately and swiftly.
Output Capabilities are highly accurate, short-term demand forecasts. These forecasts immediately adjust production schedules, inventory levels, and procurement strategies.
The goal is to align supply chain operations closely with actual market demand, thereby reducing the risks of overstocking and understocking.
Why is Demand Sensing Important?
Demand sensing is important because it provides businesses the tools to remain agile and responsive in a volatile market.
This adaptability helps companies manage their resources more efficiently and provides a substantial competitive advantage by enabling them to make informed decisions swiftly and accurately.
The modern market is characterized by rapid changes in consumer preferences and behaviors, driven by technological advancements, social media influence, and economic shifts.
Unlike traditional demand forecasting, which often relies on historical data, demand sensing incorporates the latest data from various sources, including point-of-sale systems, online sales, and social media trends.
Real-time data tracking enables planners to detect and respond to changes almost instantaneously, adjusting their strategies to align with current consumer demands and market conditions.
Competitive Importance of Demand Sensing
Integrating demand sensing strategies profoundly impacts a company’s competitive advantage.
Utilizing up-to-the-minute information and cutting-edge analyses helps businesses make judgments that immediately and accurately impact their bottom line.
This technology offers many benefits, including the ability to anticipate variations in market trends. Demand sensing can also allow companies to adjust inventory levels in a timely fashion, avoiding surpluses or shortages.
This flexibility fosters happy customers by eliminating excess overhead costs and reducing the risk of rushed shipping.
Not only this, but the speed at which businesses become aware of market changes and adapt sets them apart, exposing them to exciting opportunities ahead of their competitors.
How Does Demand Sensing Work?
Demand sensing works by continuously collecting and analyzing various data sources to provide real-time insights into consumer demand. These insights are used to make immediate adjustments to supply chain operations.
Here’s a breakdown of each step of demand sensing:
Step 1: Data Collection
The initial step in the demand sensing process is the collection of a diverse array of data. This includes real-time sales data from point-of-sale (POS) systems, online transactions, and inventory levels.
External data such as weather forecasts, social media trends, and economic indicators are also gathered.
This comprehensive data collection allows businesses to capture a detailed and current snapshot of market conditions and consumer behavior, which is crucial for accurate demand sensing.
Step 2: Analysis and Interpretation
Once data is collected, it undergoes rigorous analysis using advanced analytics and machine learning algorithms.
These technologies can process large volumes of data quickly and identify patterns that may not be visible to human analysts.
For instance, machine learning models can detect subtle changes in consumer purchasing behavior triggered by weather changes or social media trends.
This step is critical as it transforms raw data into actionable insights, enabling businesses to predict short-term demand accurately.
Step 3: Implementation
The insights gained from demand sensing are immediately implemented into supply chain decisions. This rapid application sets demand sensing apart from traditional demand forecasting methods.
Based on real-time demand insights, businesses can adjust their inventory levels, optimize production schedules, and rearrange logistics to meet current demand.
This proactive approach helps maintain optimal stock levels, reduce the risk of overstocking or stockouts, and ensure that production resources are utilized efficiently for maximum profitability.
What are the Benefits of Demand Sensing?
The benefits of demand sensing are significant enhancement of supply chain operations, improved forecast accuracy, reduced inventory costs, and boosted customer satisfaction.
Here’s a breakdown of demand sensing benefits for supply chains and manufacturers:
Improved Demand Forecasting
Demand sensing primarily improves the accuracy of demand forecasting, especially in the short term, because it utilizes real-time data from various sources.
This real-time approach allows businesses to respond more effectively to immediate market changes, reducing traditional methods’ typical forecast error rates.
Reduced Inventory Costs
One direct benefit of increased forecast accuracy is the reduction in inventory costs. Better forecasting enables businesses to optimize their inventory levels, ensuring they have enough stock to meet demand without overproducing.
This balance minimizes the costs associated with excess inventory, such as storage and obsolescence, and reduces the likelihood of stockouts that could lead to lost sales.
When inventory is closely aligned with actual demand, companies can operate more leanly and efficiently, leading to significant cost savings.
Enhanced Customer Satisfaction
Demand sensing also plays a crucial role in enhancing customer satisfaction and loyalty. Accurate forecasts ensure that products are available when and where customers need them, preventing stockouts and ensuring timely delivery.
This reliability can greatly improve the customer experience, increasing customer loyalty and repeat business.
Furthermore, the ability to quickly adjust to changing market conditions means that companies can better meet customer expectations, even in volatile markets.
What are the Challenges of Demand Sensing?
The challenges of demand sensing are data quality, the complexity of system implementation, and high-cost investments.
Businesses considering demand sensing must plan meticulously, ensuring they have the right strategies and resources to overcome these hurdles and fully capitalize on the technology’s potential.
Data Quality and Availability
A fundamental challenge in demand sensing is ensuring the quality and availability of data. Demand sensing relies heavily on inputting real-time, high-quality data from various sources.
However, collecting this data can be problematic, especially regarding consistency, accuracy, and completeness.
Issues such as data silos within an organization, outdated information systems, and the integration of external data sources can all impact the effectiveness of demand sensing.
Ensuring that data is both available and of high quality is crucial for accurate demand predictions and requires robust data management and governance practices.
Complexity of Implementation
Implementing demand sensing systems involves significant technological and organizational challenges.
Technologically, demand sensing requires the integration of advanced analytics, machine learning algorithms, and often complex IT infrastructures.
These technologies must be seamlessly integrated with existing systems such as ERP, SCM, and CRM platforms, which can be complex and resource-intensive.
Organizationally, demand sensing necessitates a shift in how teams operate, demanding close collaboration across IT, operations, and sales departments.
This complexity can lead to resistance from staff accustomed to traditional forecasting methods, and managing this change is a critical aspect of successful implementation.
Cost
The investment required for implementing demand sensing can be substantial. Advanced analytics platforms and the necessary technology infrastructure to support real-time data processing and machine learning are typically costly.
Additionally, the expenses related to training staff and hiring new talent with the requisite skills can add to the overall cost.
These costs can be a significant barrier to adopting demand sensing technology for many organizations, particularly small—to medium-sized enterprises.
Companies must carefully consider the potential return on investment and whether the benefits of demand sensing justify the initial and ongoing costs.
What are the Differences Between Demand Sensing and Demand Forecasting?
The difference between demand sensing and demand forecasting is that demand sensing focuses on the short-term horizon and uses real-time data to make immediate adjustments.
This approach allows businesses to respond quickly to market changes, making them more agile and accurate in responding to consumer demand.
Time Frame and Focus
Demand sensing is specifically designed to address the immediate, short-term changes in demand, often focusing on a horizon of days or weeks.
This approach contrasts traditional demand forecasting, which typically looks at longer-term trends spanning months to years.
The short-term focus of demand sensing is crucial for industries where demand can shift rapidly due to various factors such as promotions, weather changes, or social trends.
Data Utilization
Another key difference lies in the types of data utilized. Demand sensing relies heavily on real-time data inputs from various sources, including point-of-sale information, weather updates, social media trends, and geopolitical events.
This real-time data allows companies to adjust their forecasts and supply chain operations dynamically as new information becomes available.
While historical data is effective for understanding long-term trends, this method can be less responsive to sudden market changes, leading to potential inaccuracies in the short term.
Focusing on the immediate future and utilizing a wide array of real-time data enables demand sensing, which allows businesses to fine-tune their supply chain responses with precision and speed not achievable with traditional forecasting methods.
What are Demand Sensing Methods?
Demand sensing methods are a variety of techniques and strategies that leverage advanced technologies and traditional statistical methods to analyze and predict customer demand in real time.
These demand sensing methods are crucial for businesses looking to enhance their responsiveness to market changes and improve supply chain efficiency.
Machine Learning and AI
Machine learning and AI are at the forefront of modern demand sensing techniques.
These technologies process large volumes of data to identify patterns and accurately predict future demand. Machine learning algorithms can adapt over time, improving forecasts based on new data and outcomes.
Demand sensing uses machine learning to optimize inventory levels, predict product demand during promotions or seasonal peaks, and respond to sudden market shifts.
For instance, a retailer might use AI to adjust stock levels dynamically across stores based on real-time sales data, weather forecasts, and local events.
Statistical and Analytical Techniques
In addition to cutting-edge AI tools, demand sensing also relies on more traditional statistical and analytical techniques.
These methods provide a solid foundation for understanding demand patterns and can be particularly effective when combined with machine learning models.
Here’s a short breakdown of statistical and analytical techniques:
- Regression analysis estimates the relationships among variables. Demand sensing is used to understand how price changes, marketing campaigns, and economic conditions affect demand.
- Pattern recognition involves identifying recurring patterns or trends in data. In demand sensing, pattern recognition can help detect regular fluctuations in demand, such as those caused by seasonal changes or recurring promotions.
- Clustering algorithms group sets of objects so that objects in the same group are more similar than those in other groups. In demand sensing, clustering can categorize products with similar sales patterns.
How does AI influence Demand Sensing?
Artificial Intelligence significantly enhances demand sensing with faster and more accurate data processing and decision-making.
Implementing AI Demand sensing strategies enable businesses to analyze vast amounts of real-time data quickly, identify patterns, and make informed predictions, which are crucial for effective demand sensing.
This capability detects subtle demand signals that human analysts or traditional statistical methods might overlook.
AI systems can continuously learn and adapt, improving accuracy over time-based on new data and outcomes. This dynamic adaptation helps businesses stay responsive to market changes with precision.
Demand Sensing with Throughput
ThroughPut’s demand sensing software revolutionizes supply chain management by harnessing the power of AI and machine learning.
This technology enables businesses to accurately forecast near-term demand and dynamically optimize their supply chain operations.
ThroughPut provides actionable insights, allowing companies to swiftly adapt to market changes and align their inventory, production, and procurement strategies accordingly.
Integrating ThroughPut’s solution into existing systems offers a seamless transition to more efficient supply chain management.
Its comprehensive approach focuses on demand prediction and ensures operational alignment to reduce costs and improve product availability.
ThroughPut equips supply chain professionals with the tools to navigate modern market complexities, ensuring they stay ahead in a competitive landscape.