Building the Business Case of AI in Supply Chain Management | AI Buyer’s Guide
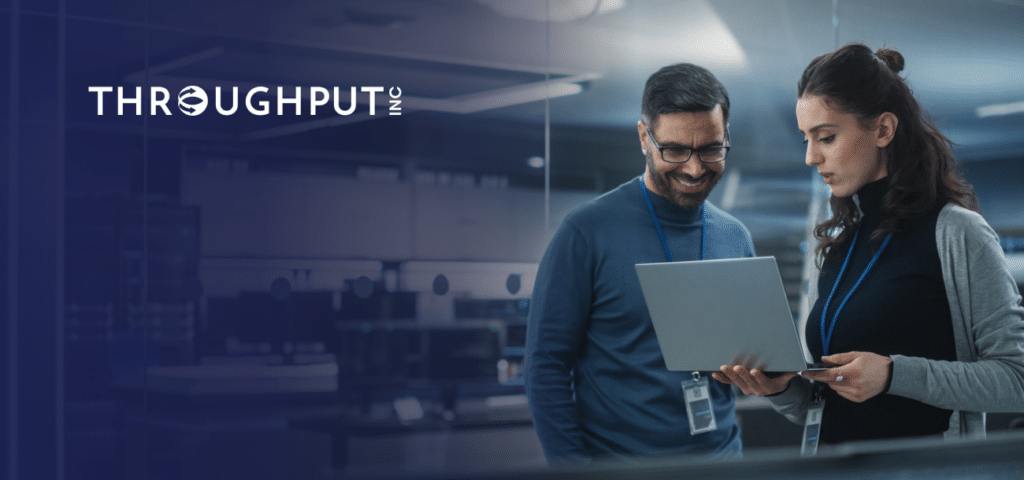
Navigating AI in supply chain management can feel like wading through a sea of contradictions.
On one hand, you hear about supply chains achieving transformative results with AI; on the other, an estimated 85% of AI projects fail. This failure rate poses a significant financial risk if those investments flop. At the same time, delaying AI adoption also has its risks, potentially leaving you struggling to keep up later.
AI is undeniably potent when applied correctly, driving efficiencies across industries from healthcare to logistics. With its potential, the AI market is expected to grow exponentially.
The key lies in implementing AI effectively.
Early adopters of AI in supply chain management have improved logistics and inventory management significantly, demonstrating the strategic advantage of AI.
If you’re a decision-maker in supply chain and looking to future-proof your supply chains, it’s crucial to leverage AI wisely to enhance resilience and operational efficiency.
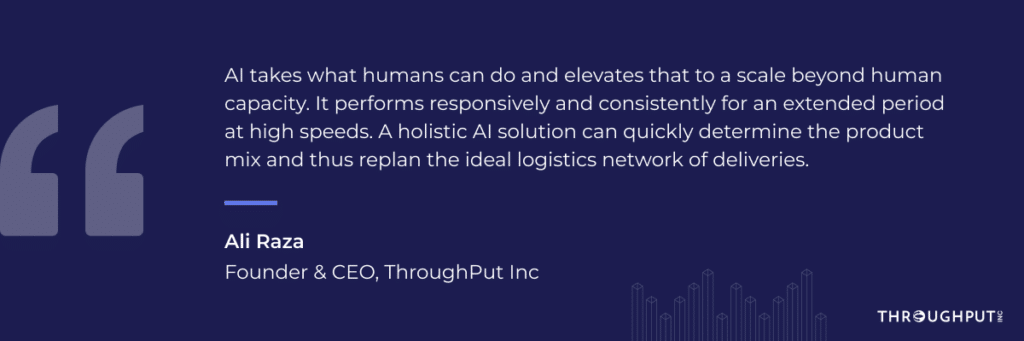
This guide is designed to help you establish the business case of AI in supply chain without delving into the technicalities meant solely for data scientists or software developers.
Building the Business Case of AI in Supply Chain Management
There are some critical realities of the business landscape today that necessitates AI in supply chain.
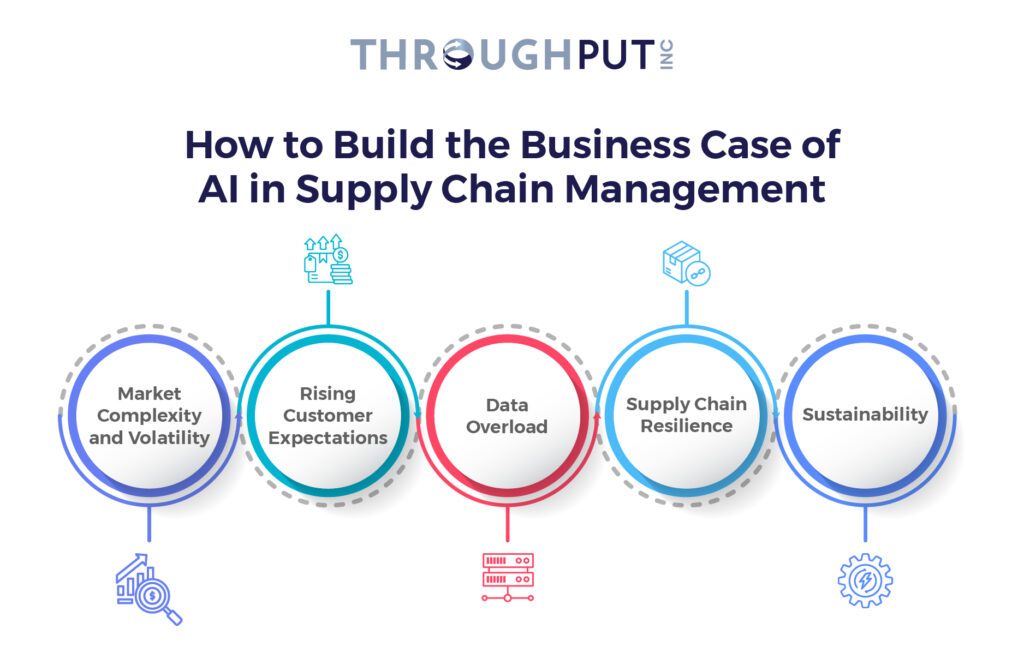
- Market Complexity and Volatility: Global markets are becoming increasingly complex and unpredictable, driving the need for adaptive supply chain solutions that can respond swiftly to changing conditions.
- Rising Customer Expectations: As customer demands reach unprecedented levels, real-time visibility and actionability becomes essential for ensuring that your business remains responsive and competitive.
- Data Overload: The explosion of data presents a significant challenge, but it also offers an opportunity. With advanced analytics, you can turn this data into actionable insights that drive better decision-making.
- Supply Chain Resilience: In a world where disruptions are more frequent and severe, building a resilient supply chain is critical for maintaining continuity and mitigating risks.
- Sustainability: Today, sustainability isn’t just a buzzword—it’s a fundamental requirement. Efficient resource utilization is necessary to meet environmental and regulatory demands.
Integrating AI-driven technologies into your supply chain operations is not just an option anymore—it’s a necessity to thrive and sustain in today’s rapidly evolving business landscape.
Supply Chains Are Being Tested. Are You Ready to Respond?
Traditional supply chain methods can’t keep up with today’s complex and fast-changing landscape. Manual processes, outdated technologies, and disconnected data systems expose your business to significant risks and inefficiencies. To stay competitive, you need to rethink your approach and close the gaps in your processes, people, data, and technology.
Adopting modern, data-driven strategies is key to building a supply chain that’s not just efficient but resilient and adaptable. By embracing new technologies like AI, you can better predict customer demands, optimize inventory, and allocate resources more effectively. This approach empowers you to respond quickly to changes, manage alternative suppliers, and navigate growing logistical challenges. In a world where traditional methods fall short, leveraging AI and other advanced tools is crucial to turning vulnerabilities into opportunities and ensuring your supply chain thrives.
🏃♂️➡️ Action Tip: Close the Gaps
Before diving into AI adoption, it’s crucial to close any gaps in your organization’s foundation.
Start by assessing whether your processes, people, data, and technology are ready to support AI. If you identify clear weaknesses—like outdated systems, insufficient data, or a lack of skilled talent—focus on addressing those. By building a strong foundation, you ensure that your AI initiatives will be more successful and sustainable in the long run. Trying to implement AI without this groundwork can lead to inefficiencies, setbacks, and missed opportunities.
Supply Chains Are Evolving. Can You Keep Up?
Supply chains are evolving rapidly, and organizations like yours are increasingly focused on automating workflows and integrating AI to stay competitive. This shift is driven by the need to incorporate granular, real-time data into every aspect of supply chain operations. As business environments shift and consumer behaviors change, the demand for flexibility in decision-making and production planning grows. In this dynamic landscape, AI is essential for staying ahead. By analyzing vast amounts of data, AI technologies offer predictive analytics that deliver actionable insights, enabling your business to adapt and thrive amid these changes.
🏃♂️➡️ Action Tip: Be Clear on the ROI
Before committing to AI, it’s essential to assess its potential return on investment. Begin by asking these fundamental questions:
- What specific challenge in your supply chain will AI help address?
- What tangible benefits will arise from resolving this challenge?
- What makes the AI solution more effective than simpler alternatives like manual methods?
These questions will help clarify the relevance of AI for your particular needs and guide you in tracking the most appropriate business metrics.
Clearly evaluate the value that AI brings to your business challenge. If the benefits are marginal or if simpler solutions could achieve similar results with less complexity, AI might not be the right choice. Prioritize AI only when it offers significant improvements and clear, measurable advantages.
Digital Transformation Is Not Possible Without a Data-first Approach
For any successful digital transformation in supply chain management, a data-first approach is essential. This focus on data drives recovery, growth, and innovation, ensuring that your supply chain adapts effectively to change. While digital transformation is important, it’s the emphasis on data that enables practical, agile, and impactful adoption of automation and AI. A data-first strategy ensures your transformation efforts go beyond surface-level changes, building resilience and stability across your entire supply chain.
Key Benefits of a Data-first Approach:
- Establishes data quality, lineage, and transparency
- Enhances data accessibility for informed decision-making
- Facilitates rapid data delivery for timely analytics
- Produces relevant and actionable data quickly
- Supports vital business use cases
- Promotes strong governance and compliance practices
🏃♂️➡️ Action Tip: Remove Bias from Data
When implementing AI in your supply chain, it’s crucial to address potential biases that could impact decisions and outcomes. To do this effectively, you and your team should consider the following questions:
- Where does the data you’re using to train AI models come from?
- What biases are already known to exist in this data?
- What could be the consequences of these biases in your supply chain? Could they lead to unfair supplier evaluations, incorrect demand forecasting, or biased resource allocation?
- Can these biases be eliminated, and is it worth the effort to do so?
By asking these questions, you can guide your team in identifying and mitigating potential biases. While some issues may be resolved by closely examining your data, others will require a deeper, more thoughtful approach.
AI Can Build Supply Chain Resilience
CIOs and business leaders are increasingly focused on strengthening supply chain resilience to better adapt to shifting demand patterns and evolving e-fulfillment needs. By enhancing the speed and resilience of your supply chain, you can effectively address new competitive threats and prepare for future challenges. Automated, end-to-end capabilities are crucial for building robust digitization plans, especially in uncertain times, driving both maturity and performance.
Artificial Intelligence is pivotal in this process. AI algorithms and machine learning provide real-time insights, giving decision-makers greater visibility across the supply chain. Predictive analytics allow for more accurate forecasting, enabling you to anticipate disruptions and proactively manage risks. By reducing reliance on manual processes and traditional forecasting methods, AI streamlines operations, making your business more agile and responsive to change. This adaptability is essential for maintaining a resilient supply chain that can thrive under any circumstances.
🏃♂️➡️ Action Tip: Evaluate AI Solutions Thoroughly
While prepackaged AI solutions might appear to be a straightforward choice, it’s crucial to determine if they truly align with your business needs. These off-the-shelf tools are often built to be broad and adaptable, which can result in suboptimal performance when applied to your specific data or operational environment. Common issues you might encounter include inaccuracies, slow response times, and difficulties in integration.
To ensure that a prepackaged AI solution is the right fit, ask the vendors the right questions:
- Can the software scale out as well as in?
- How long will it take to demonstrate ROI?
- How does the solution integrate with other software and tools?
- Is the software user-friendly?
- Does the software adequately support automation and customization?
- Does it have data security in place?
Begin by also clearly defining your AI problem, as this clarity will help you measure the solution’s effectiveness.
What’s Holding You Back?
Supply chain networks generate enormous amounts of data, leading to significant challenges that can disrupt decision-making across sourcing, manufacturing, distribution, and logistics. These complexities often result in inefficiencies, delays, and rising operational costs. Traditional methods may struggle to manage this data effectively, leaving your supply chain vulnerable to reduced agility and responsiveness. Overcoming these obstacles is essential to maintaining a competitive edge and ensuring that your supply chain can adapt to changing market demands, something that AI can do for you.
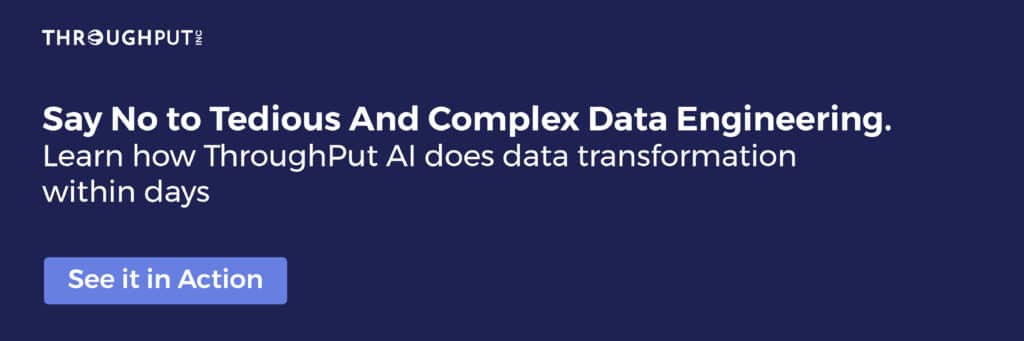
🏃♂️➡️ Action Tip: Measure Success
To ensure the success of any AI initiative, it’s essential to focus on three foundational pillars:
- The AI model’s performance, both during development and in real-world production environments.
- How well the AI solution aligns with and advances the organization’s strategic objectives.
- The satisfaction of end-users and their perception of the AI as a credible and effective solution.
Challenges to Implementing AI in Supply Chain
Implementing AI in supply chains is crucial but far from straightforward. The adoption process can be challenging, especially if quick fixes are applied without addressing underlying issues. These “magic” solutions often exacerbate existing problems, making it harder to achieve long-term success with AI and analytics. True transformation requires a thoughtful approach, starting with identifying and addressing data gaps and inconsistencies. By effectively leveraging data, you can drive innovation, uncover new opportunities, enhance operational efficiencies, and fundamentally reshape the way your organization operates. This deliberate and strategic approach is essential for realizing the full potential of AI in your supply chain.
Read More: The 12 Challenges of Implementing AI in Supply Chain
🏃♂️➡️ Action Tip: Ready Your Organization for AI in Supply Chain
Cultivate a culture that actively embraces change and prioritizes data-driven decision-making. Leaders need to take charge by promoting AI initiatives, fostering cross-department collaboration, and empowering teams to leverage AI insights effectively. Address resistance head-on by clearly communicating how AI enhances human capabilities rather than replacing jobs. Focus on building a culture that is ready and willing to adapt, ensuring that AI integration not only happens smoothly but also delivers immediate and lasting value to your supply chain operations.
Data Preparation for AI Implementation in Supply Chain
Unlocking the full potential of AI in your supply chain requires robust data preparation. Effectively harnessing the power of data is essential for addressing challenges and uncovering new opportunities. Real-time data feeds are crucial for continuously monitoring, evaluating, and refining your supply chain strategies, implementations, and performance. Key sources of data include internal systems, external market data, supplier inputs, and IoT-generated data.
🏃♂️➡️ Action Tip: Ask the Right Questions for Data Preparation
- Do you have the right data available in an accessible and optimal format?
- What data processing steps are necessary to make the data usable?
- Can you identify and address data gaps?
- Are the appropriate data authorizations in place?
- Is there a clear connection between data authorization and accountability?
- Can your data be seamlessly integrated with existing processes and use cases?
Read More: How ThroughPut AI Achieves ROI in 90 Days?
Case Studies of AI Implementation in Supply Chain
Case Study 1: Minimizing Unplanned Machine Downtime
The Challenge: A large industrial operation with over 100 facilities was struggling with frequent unplanned machine downtime, aiming to reduce associated costs by $500,000 annually.
Solution: Using Throughput’s AI-powered tool, the company connected existing sensor data and introduced additional sensors to monitor industrial equipment uptime. The tool compared downtime events with historical data, identifying root causes and quantifying monetary losses.
Results:
- Identified root causes of unplanned downtime
- Achieved a 5% reduction in unplanned downtime through real-time monitoring
- Realized $1.5 million in cost savings
Case Study 2: Carrier Optimization for Trucking Brokerage
The Challenge: A technology-driven trucking brokerage with a fleet of over 9,000 trucks struggled to gain real-time insights into profits and performance, impacting its ability to match top carriers with the most profitable customers.
Solution: Throughput AI was employed to analyze transportation management system data, identifying the most profitable and high-performance customers. The AI software also provided insights into improving customer service and reducing delivery delays through route optimization.
Results:
- Flagged over 50% of deliveries that were causing financial losses
- Identified the top 5 carriers to pair with the best customers
- Achieved an 80x return on investment (ROI)
Case Study 3: Retail Operations Optimization
The Challenge: A leading retail chain faced challenges with inventory overstock, waste, and logistics inefficiencies due to a lack of insight into its best and worst-selling products.
Solution: Throughput’s AI-powered tool analyzed key performance indicators (KPIs) such as labor productivity per employee, inventory turnover by SKU, shipping costs, and lead times for premium products.
Results:
- Simplified the supply chain by eliminating non-performing products
- Achieved a 5% increase in labor productivity
- Reduced inventory by 15%
- Eliminated 28 unnecessary product modifiers
These case studies illustrate the transformative impact AI can have on supply chain operations, driving efficiency, reducing costs, and improving overall performance.
What Next: Integrating AI into Supply Chain Operations
To succeed with AI, think big but start small.
Begin by implementing AI in specific areas of your supply chain where you can easily measure results and preferably with the most impact, such as inventory management or demand forecasting. This approach allows you to test and refine AI solutions without overwhelming your resources.
Read: AI Use Cases in Supply Chain
As you gain experience and see positive outcomes, you can gradually expand AI applications to other parts of your supply chain. By keeping your long-term goals in mind, you ensure that each small step builds towards a comprehensive, AI-driven transformation. This balanced strategy helps you manage risks, learn from each phase, and ultimately achieve significant improvements across your entire supply chain.
There’s no one-size-fits-all solution for supply chain transformation. However, certain practices can help you make the most of digital technologies. Start by setting up strong data preparation processes. Use a fast, iterative approach that allows quick retraining with new data. This will keep your AI models accurate and up-to-date.
Focus on a human-first approach. Enhance your team’s capabilities by integrating their daily challenges into AI workflows. Choose simple, sustainable solutions that build trust in AI. Make sure your supply chain transformation aligns with broader business goals. Avoid random automation. Instead, automate carefully to create seamless, end-to-end processes that connect people, technology, and operations.
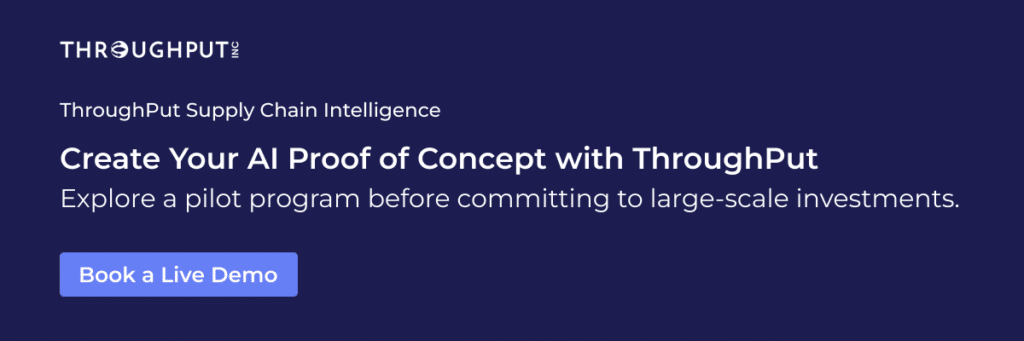