AI-Driven Supply Chain Decision Intelligence: Making Smarter and Faster Supply Chain Optimization a Reality
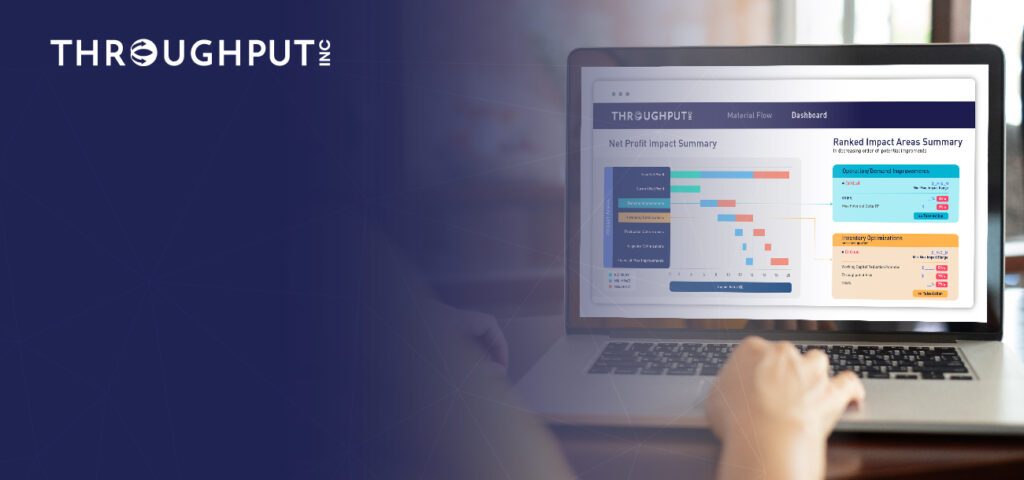
Business success depends on decisions – decisions that range from target market selection to vendor engagement, production and sales targets, logistics routes, and even talent hiring.
However, decision making is plagued with challenges, and to make things worse, complex and critical decisions are often made by people with severely inadequate information, time, or experience.
Unsurprisingly, the average Fortune 500 company wastes about $250 million in wages and 530,000 days in managers’ time every year as a result of ineffective decision-making.
The supply chain too, like every other part of an organization, is essentially a series of well-informed, intelligent decisions – when done right, forming the backbone of every successful business. However, making these decisions is far from straightforward, as every executive will vouch for.
Supply chain teams are now transitioning into yet another year amidst continued supplier, part, and logistics disruptions that have challenged teams across the world since the onset of COVID 19.
As a result, making business decisions requires significant efforts in gathering and analyzing data. This often involves scrutinizing spreadsheets or similar legacy applications – leading to an inherently retrospective approach to decision-making.
Businesses, however, need real-time, relevant, and actionable intelligence to effectively manage increasingly complex supply chain networks. Without key, timely, and holistic supply chain decision intelligence, they run the risk of flawed, siloed decisions, process and operational gaps, and lost sales and revenue.
What’s more, increasingly frequent disruptions from the COVID-19 pandemic to semiconductor shortages, the Suez Canal crisis, and the Ukraine war have bared the fragility of supply chains around the world.
As a result, businesses are now confronted with the need to rethink and transform foundational assumptions about supply chains – and how they plan, forecast, and operate them.
What Exactly is Supply Chain Decision Intelligence?
According to Gartner, decision intelligence is “a practical domain framing a wide range of decision-making techniques bringing multiple traditional and advanced disciplines together to design, model, align, execute, monitor and tune decision models and processes.
Those disciplines include decision management (including advanced nondeterministic techniques such as agent-based systems) and decision support as well as techniques such as descriptive, diagnostics and predictive analytics.”
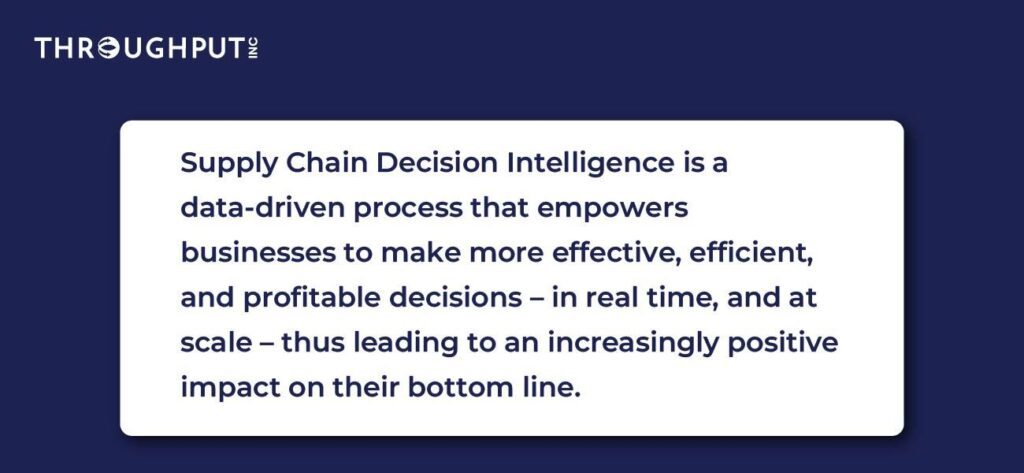
Similarly, supply chain decision intelligence is a data-driven process that empowers businesses to make more effective, efficient, and profitable decisions – in real time, and at scale – thus leading to an increasingly positive impact on their bottom line.
Supply chain decision intelligence enables businesses to function rapidly, intelligently, and efficiently to achieve and more importantly optimize their business goals.
Why is Decision Intelligence Important in the Supply Chain?
Supply chain operations generate oceans of diverse data about customer preferences, trends, order changes, production operations, inventory levels, and logistics routes and costs.
This data can generate tons of valuable insights to recommend decisions about demand fulfillment, production load balancing, logistics cost optimization, and more.
Such decision intelligence enables businesses to make accurate, effective decisions – rapidly, efficiently, and at scale – regardless of changing business dynamics.
With better access to actionable intelligence, stakeholders can generate millions of decision-making alternatives and evaluate “what if” scenarios and their associated trade-offs.
More importantly, coupling decision intelligence with the operational and regulatory constraints of supply chains helps executives hone their choices and make smarter decisions.
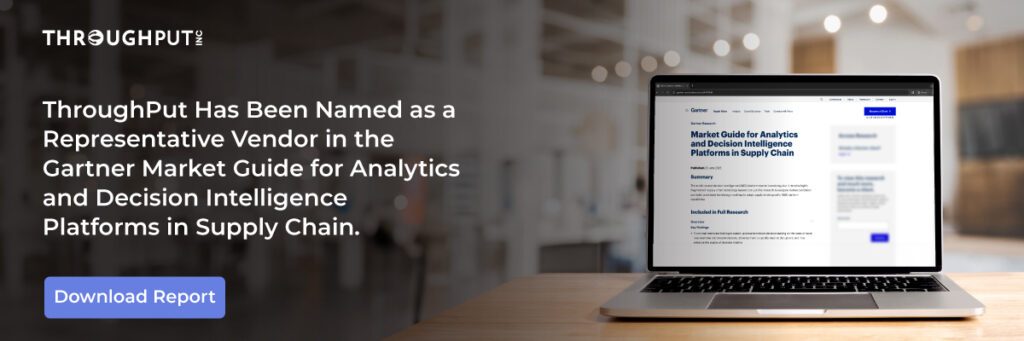
How Does Decision Intelligence Work?
According to Gartner, there are three levels at which supply chain decision intelligence can support business decisions.
- Decision support: The first level is decision support, where machines provide some basic features – such as alerts – to support decision-making. The decisions, however, are made entirely by humans.
- Decision augmentation: The second level of supply chain decision intelligence is decision augmentation, where machines play a more proactive and significant role in the decision-making process. They analyze data to generate predictions and recommendations that human decision makers can review and validate. If, for example, a recommendation to buy 500 units from vendor A two times a year to enable end-year savings of $35,000 is made, executives may choose to accept the recommendation, or they may amend a few of the variables to evaluate other potential scenarios and outcomes.
- Decision automation: The third level is decision automation, where the human involvement required in the decision process further reduces. At this level, machines have the autonomy to decide as well as execute. Decisions are made as a result of AI-based rules, predictions, and optimizations. They are then implemented automatically, freeing up executives’ time as they are only required to monitor any unusual activity or potential risks, and regularly review outcomes to improve the system.
An effective supply chain decision intelligence system should offer all three levels of intelligence – support, augmentation, as well as automation – to enable businesses to develop trust in the system and progress up the levels of automation.
The Role of AI in Supply Chain Decision Intelligence
AI is what makes supply chain decision intelligence ‘real-time’ ‘fast’ and ‘accurate’.
With AI, businesses can make sense of large volumes of data faster and in real-time, automate decision making, minimize risk with greater accuracy in decisions, and identify and leverage opportunities – at scale, every day.
According to a BCG report, “Planners need to understand the insights they receive, but typically they do not have enough time to digest all the inputs and make good decisions.” The report recommends shifting toward a new approach of piloting automation tools over mere visibility and analytics, which will be powered by AI.
The potential growth opportunities that result from an AI-powered supply chain intelligence engine can be game-changers for any business – driving greater profits by prioritizing customers and SKUs with greater value and demand, aligning with more reliable suppliers to enable better OTIF performance, or sensing demand in real-time to streamline upstream activities – leading to continuously incremental improvements on the bottom line.
The window of opportunity to make the decisions in supply chain is usually small. It may take hours or even days for teams to analyze the necessary data, align on a plan of action, and execute it – assuming that they can even have the visibility and bandwidth to recognize the opportunity altogether. AI-powered supply chain decision intelligence bridges this gap for good.
The Difference Between Business Intelligence and Decision Intelligence
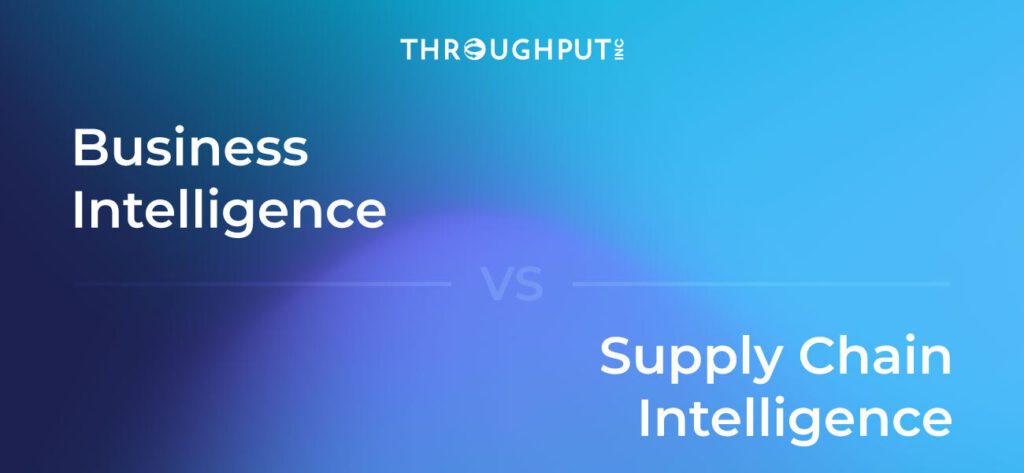
The key difference between business intelligence and decision intelligence is in the type of intelligence that each provides. Business intelligence is the process of enabling the visualization of operational results in various formats such as dashboards and reports.
Decision intelligence, on the other hand, empowers executives with insights and recommended actions that they need to solve business problems.
For example, business intelligence may indicate by how much sales increased or decreased over the last quarter or year, while decision intelligence drills down further to explain the reason for the change, and also to help either further accelerate the increase in sales, or solve the challenges causing sales to decline.
If total sales increased due to a campaign exceeding its targets, or total sales went up despite sales actually slowing down across 73% of business locations, business intelligence would simply show the increase in sales – meaning that if business were to go on as usual, in the latter scenario, performance could decline.
Decision intelligence, on the other hand, would drill down the results to explain why sales went down across a majority of locations, and recommend the necessary actions to improve it – this intelligence could then be shared with all other stakeholders to ensure corrective action across the board.
In other words, supply chain decision intelligence provides a much more thorough understanding of business performance, thus enabling amplification of positive outcomes.
Examples of Supply Chain Decision Intelligence
Decision Intelligence for Capacity Planning
In the capacity planning space, supply chain decision intelligence involves leveraging data from Enterprise Resource Planning (ERP) systems – as well as other data sources, including third-party sources – to support a wide range of use cases such as optimizing capacity, identifying and eliminating bottlenecks, prioritizing the right product mix for production and meeting demand accurately.
This enables businesses to effectively optimize and manage capacity by implementing a continuous, demand-driven feedback loop that ensures adequate capacity and optimal inventory levels at all times, taking into account actual capacity as well as real-time market dynamics.
Let’s take the example of an Aerospace sensor company. With a highly complex, 50+ step manufacturing process, ramping up production became a massive challenge for this business, with manufacturing taking place at a slower pace and in lower batches than required.
Without visibility into this complex process, identifying the areas for improvement and the actual scope for improvement was becoming a growing challenge for this aerospace sensor company.
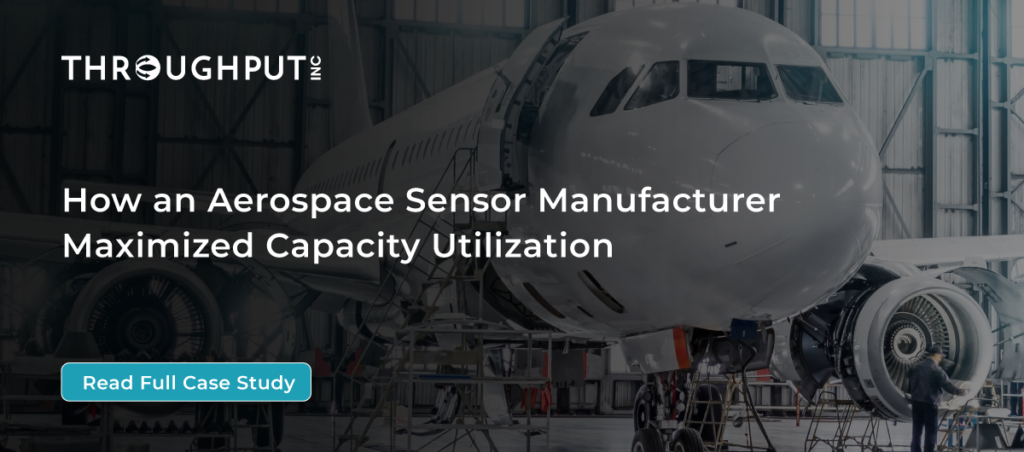
Using AI in supply chain and logistics, they were able to bring granular visibility into their complex production process and identify undetected bottlenecks and underutilized capacity, among other things, accelerating their operational productivity by 20%, leading to a yearly savings potential of $10 million and a 10% reduction in processing time.
Decision Intelligence for Logistics Management
When it comes to logistics, many businesses struggle to determine when to ship, how many vehicles to use, and which routes to use to minimize costs, support ongoing capacity, and deliver on time and in full (OTIF).
With the right decision intelligence, they can leverage cognitive predictions and recommendations to optimize all these operations, manage fluctuating demand, and thus prevent over or under ordering vehicles – which means that they neither sit idle nor are insufficient for order fulfillment.
Either way, decision intelligence helps prevent loss of revenue by predicting variance and accordingly offering real-time improvement recommendations aimed at identifying and eliminating bottlenecks and maximizing the efficiency of logistics operations.
Let’s understand better with an example.
Ready Mix Concrete is a tricky industry as far as the supply chain is concerned because the product, the ready mix concrete, can only be prepared on request as it solidifies very quickly. This means that production scheduling and truck dispatching need to happen like clockwork to maximize revenue and achieve customer satisfaction. In the case of a leading Ready Mix Concrete (RMC) company, achieving maximum efficiency while meeting sustainability goals was a top priority.
Using supply chain decision intelligence capabilities, they analyzed existing data from multiple sources and:
- Developed a 360-degree customer scorecard taking into account multiple variables, including number of jobs, sales volume, load size, average distance to plants, and recall percentage to segment customers and suggest the most optimum customer mix.
- Suggested opportunities to enhance asset utilization by identifying why trucks were not sent to the closest plant, analyzing the reason for high return travel times, reducing customers with high on-site times, and more.
Decision Intelligence for Sustainability
Supply chain decision intelligence plays a crucial role in accelerating sustainability in several ways. It helps companies, which typically lose millions of dollars a year – as a result of wastage due to expired material, outdated packaging, etc. – reduce emissions as well as costs by detecting and mitigating risk of wastage through enhancing operational efficiency.
With supply chain decision intelligence, these companies can leverage demand and supply, shelf life, and other relevant data to predict the risk of wastage and identify the right procurement, production, and/or logistics actions to mitigate it.
Examples of recommended actions may include:
- Updates to demand forecasts
- Promotions aimed at clearing stock at risk
- Identifying the right purchase orders to process so that order fulfillment can be optimized (and wastage minimized)
- Inventory transfers to balance input and output
- Updates to production schedules
Decision intelligence can also help minimize CO2 emissions by optimizing logistics routes based on analyzing the trade-offs between cost, emissions, and OTIF deliveries (quality of service).
During the COVID-19 pandemic, many businesses fulfilled orders via air to overcome clogged sea routes and avoid delays, thus meeting customer expectations and preventing missed sales. However, sending freight by air rather than rail, road, or sea results in a high impact on carbon emissions.
Supply chain decision intelligence helps businesses analyze order, inventory, transport, and emissions data – and factor in planned vs actual lead times – to calculate “what if” scenarios for different cost, emissions, and service levels for every order – and identify opportunities to optimize order fulfillment so that emissions are minimized.
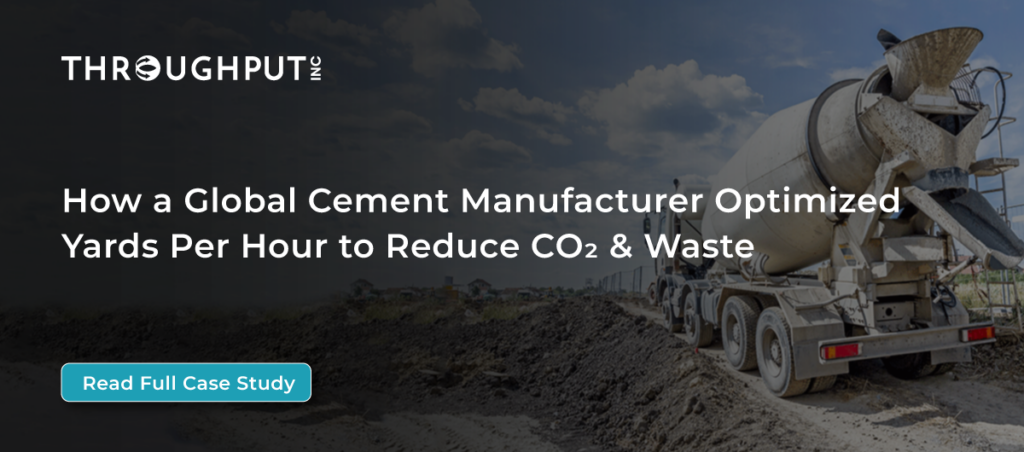
Finally, decision intelligence can help select alternate suppliers to ensure more sustainable procurement of material.
For example, it can help choose between different suppliers (that use virgin plastic vs. recycled plastic, for instance) by analyzing usage against sold product, wastage, cost, supplier performance, and sustainability impact to select the best supplier at a given time.
Decision Intelligence for Demand Sensing
This is an area where decision intelligence can really be transformative – it helps understand demand signals in near-term so that businesses can accordingly align production, dynamically price products to maximize sales, and deliver the right product to the right place at the right time. It helps businesses manage supply chain disruptions, move with agility, and match supply to changing/increasing demand by eliminating siloed data and traditional demand management processes that were typically reactive rather than proactive.
Let’s look at the example of a US-based agribusiness. As a fresh produce brand producing vegetables with limited shelf life, ensuring the right products in the right quantity are available at the right times was super critical for this business.
With 60 commodities, 25 value-added products, and 490 product groups being shipped in 50 million cartons and with 4,400 truck deliveries annually – they had a complex supply chain that urgently required granular visibility and actionable recommendations to optimize everything upstream as per demand.
Using decision intelligence capabilities, they uncovered critical insights and recommendations around:
- How often over the past period and year has a particular customer expressed purchase interest?
- How many equivalent cartons has this customer purchased, and will they most likely buy in the future?
- What was the overall price variance for this customer over the past year, and how does one best manage that?
- How many commodities has this customer purchased, and will they most likely purchase in the future?
- What percentage of the commodities bought were value-adds, and how much contribution margin did they account for across each customer?
Decision Intelligence for Inventory Management
Decision intelligence enables businesses to analyze historical sales data, customer behavior, and any relevant external factors such as seasonality and trends to optimize their inventory levels.
This involves identifying the right balance to avoid overstocking (that ties up capital) and understocking (that results in lost sales) so that there is just enough inventory to meet demand.
By accurately forecasting demand, supply chain decision intelligence helps retailers ensure that adequate inventory is always available to satisfy demand, while avoiding overstocking as well as stockouts.
A very relevant case study in this regard would be that of a retail coffee chain. One of the most critical things for a cafe business is its menu. Knowing what sells and what doesn’t is essential when it comes to stocking raw materials. A miss on this front can mean either wastage due to overstocking or disappointing precious customers due to understocking.
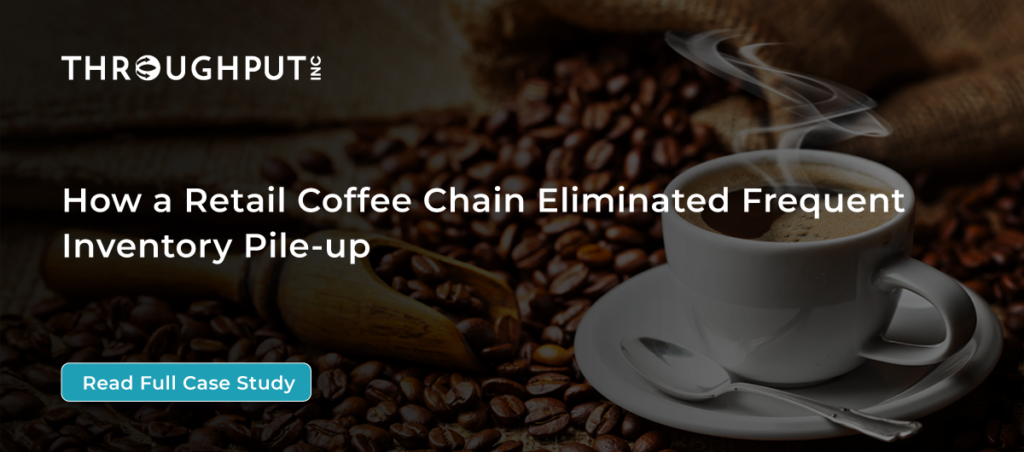
This particular coffee retailer, too, struggled with a lack of visibility into its best and not-so-best performing offerings, inventory pile-up, waste of food, and logistics issues.
The company chose supply chain intelligence to get recommendations on product prioritization based on demand sensitivity and accordingly optimize their inventory.
Decision Intelligence for Financial Management
With the right decision intelligence, businesses can reduce costs across their supply chain processes and activities, helping them minimize both their OPEX and CAPEX.
In addition, they can accelerate cash flow conversion cycles and rapidly eliminate losses by identifying top and bottom-performing products – leading to better resource allocation, logistical movement, and marketing decisions.
Thus, businesses can affect incremental improvements at a micro level along the entire length of the supply chain, ultimately achieving a significant impact on the bottom line.
ThroughPut for Supply Chain Decision Intelligence
ThroughPut’s purpose-built solution for supply chain decision intelligence enables businesses to manage and optimize activities across their entire supply chain network – right from demand and capacity planning to supply and inventory management, sales and operations planning, and distribution, logistics, and order fulfillment. Thus, businesses can effectively manage and optimize:
- Demand by segmenting product portfolios and forecasting various what-if scenarios to enable accurate demand and budget planning
- Capacity by implementing a real-time demand-capacity feedback loop that ensures just-right inventory levels at all times and takes into account ongoing capacity constraints as well as market conditions
- Inventory by ensuring a smooth flow of goods and materials into and out of warehouses at the right time, thus preventing overstocking as well as understocking – and ultimately, wastage or lost sales
- Transportation by enabling logistics managers to identify systemic bottlenecks and prioritizing fixes in a manner that maximizes net cash flow and profits
- Sales and Operations (S&OP) by enabling key decisions based on highly accurate demand forecasts and inventory levels
In other words, with ThroughPut, businesses can leverage truly data-driven decision-making that empowers them to plan for – and adapt to – the future.
In Conclusion: The Future of Supply Chains is in Decision Intelligence
As customer expectations and business models continue to evolve, the key to business success will hinge entirely on timely, intelligent, and accurate decision-making across the supply chain – which is no longer feasible or scalable if implemented manually, or based on historical data or gut instinct. The growing cadence of market disruptions is proof of that.
With AI, supply chain networks can leverage data-driven decisions aimed at anticipating and planning for the future, at every level and node.
More importantly, businesses have a single source of truth, right from planning and strategy through to execution, enabling them to significantly improve customer experiences, minimize supply chain risks, and accelerate business growth.